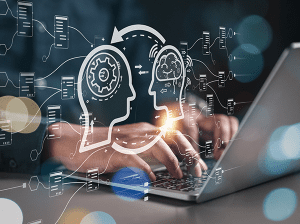
As businesses adopt generative AI, a technology that creates new content and finds patterns, what will Data Science, the processes and activity geared to getting insights from big data, look like? How will this kind of analytical work change? Will there be an increased or decreased need for data scientists?
How should businesses adapt their information science programs? What organizational resources will decision-makers need to effectively interpret data?
To learn more, DATAVERSITY® interviewed Michael Berthold, the CEO of KNIME. Berthold is a Data Science veteran of 30 years and an author of over 250 publications. He provided insights into how generative AI changes Data Science, opening new ways to solve more interesting and complex puzzles.
Changing the Data Science Profession for the Better
Generative AI functions as a customizable, super assistant tool. Berthold said, “It is a consensus model of available knowledge that is out there” and “it can result in innovations in human-generated content, like videos and text.”
For the data scientist, Berthold said, “Generative AI does the mundane tasks wonderfully.” He noted that generative AI does the following:
- Takes care of the simple details in the Data Science workflow by extracting, transforming, and loading (ETL)
- Does standardized and routine Data Science tasks
- Offers options to configure complex modules
- Improves code embeddings, which simplify the way computers process relationships between various segments of code
- Matches data sets to figure out what information fits in where
- Gets better Data Quality processes
Additionally, he explained that generative AI algorithms provide more building blocks for different generative AI codes. These features “augment what people used to do with their analytics platforms.” He included:
- Metadata or wrappers around the large language models (LLMs), the technology that predicts and generates the generative AI engine’s responses
- Connectors to different existing generative AI models
- Machine learning (ML) functionality with other modules and other code libraries
By automating routine tasks and providing additional services, generative AI can significantly reduce the preprocessing work data scientists do. This preprocessing, which involves arranging and restructuring data, can take up to 80% of a data scientist’s time. Consequently, data scientists can focus on interesting and complex problems and come up with unique insights, said Berthold.
Improving Data Science Constantly Thanks to Open Source
By the time this article is read, these AI models will demonstrate new capabilities in Data Science. Berthold said, “Free access to open-source libraries and communities, like those supported by KNIME, speed up generative AI inventions and developments.”
He added, “No proprietary software vendor has a chance to stay current in the Data Science space without relying on the open-source community. This resource helps companies catch up with the latest trends.”
Keeping the Data Scientists
Despite exciting advances in generative AI, the tool has its drawbacks. Michael Berthold emphasized, “LLMs fall flat on their face when deriving new insights and knowledge.” He added, “Generative AI tools make average insights, but business wants to find new ones from the data. These new ideas come from domain and tool expertise that humans provide.”
When the data scientist demonstrates domain expertise, they understand the basis of their analysis. They see where the conclusion “is numerically convincing, but does not seem right,” he said. These experts dig deeper, find the flaws in the data, and reach a more accurate assessment.
A data professional’s tool knowledge keeps an organization on track to a solution. Berthold said,
“Everyone is throwing generative AI elements at every single problem. That is silly. It is just another tool in the tool belt. Companies still need to understand the normal methods and technology given them and how to complement that nicely with generative AI.”
Expertise in tools brings an understanding of what these resources can potentially do and when to bring in AI technology. So, Berthold sees the demand for domain and tool knowledge as increasing. This, in turn, will drive the need for data scientists.
Filling the Data Science Void
Organizations will continue to need more data scientists to fill a void in the specialized knowledge, as described above. To do so, enterprises may think to turn to the businessperson – say, the sales professional that automates quarterly financial reports. Berthold described this worker as one who can build a Microsoft Excel workflow and reliably do the data aggregation, avoiding errors.
That employee can use an AI chat assistant to “upskill their Data Literacy,” says Berthold. They can come to the LLM with their “own perspective and vocabulary,” and learn. For example, they can get help on how to use Excel’s Visual Basic (VB) code to look up data and see what functions will be available, from there.
While LLMs make it easier to do Data Science, “at the end of the day, anyone needs to be willing to learn, know the techniques and tools to become a data scientist,” said Berthold. He observed that such a person must know what the LLM model means and where it came from.
Furthermore, he believes any businessperson in transition to a data scientist profession needs to know:
- What can be done with the datasets
- How to preprocess data for analysis
- How to use statistics
- How to use ML methods
With this knowledge, these budding professionals can learn to think about the data and avoid potential biases. “This critical thinking capability is needed now more than ever,” said Berthold.
Preventing Hallucinations
Critical thinking comes in handy when generative AI hallucinates, as it generates output that seems plausible but is incorrect, inconsistent, or nonsensical. This tendency can cause problems.
Berthold gives an example where he tried to get ChatGPT, a generative AI model, to write a Data Science introduction. He said:
“ChatGPT generated a lot of super text but left massive mistakes. These can really be hard to find because the content reads so convincingly. As a person continues processing the LLM’s response, they do not realize they are reading nonsense until they go back and question. In this case, I ended up arguing with this AI model and never really reached a consensus.”
Mistakenly interpreting a hallucination as true, which is easy to do, can lead to a biased and unhelpful decision. So, organizations need to guide and support workers to more productive activities.
Supporting Data Scientists Through Governance
Berthold advises businesses, “If a company wants people to work with Data Science and to utilize generative AI, becoming productive, then it needs a compliance framework in place.” This management requires a Data Governance component, so “that data does not accidentally leak all over the place.”
He acknowledged that organizations struggle with Data Governance, with the fear of having data sets and teams all over the world compliant. However, setting up such a program is necessary to comply with the data laws, like the GDPR, whether using generative or not.
About a quarter of companies have responded to the increased risk by implementing bans on LLM usage. But with the technology readily available, some workers will find a way to use these LLMs in their work.
So, AI and Data Governance need to put controls and safeguards in place. Berthold sees that a commercial platform, like his own company, can come in handy in supporting these requirements. He said,
“Organizations need governance programs or IT departments to only allow access to the AI tools their workers use. Companies need to ensure they know what happens to the data before it gets sent to another person or system.“
He noted that many companies anonymize data before transporting it to the cloud and then deanonymize the data on the other end, protecting privacy. This process and a strong Data Governance program can support data scientists’ productivity while being ethical and compliant.
Conclusion
Generative AI promises an exciting future, especially in Data Science. Berthold emphasized that AI engines handle simple, boring tasks and bring a greater opportunity to those wanting to upskill their Data Science skills.
In the meantime, some companies will become disillusioned with these tools, given their lack of new insights and problems with hallucinations. But here the need for data scientists with domain and tool knowledge will grow. They will select specific business problems that would benefit from generative AI and question what information they return.
With additional Data Science needs, organizations will provide Governance to use AI well and its data more objectively, while protecting privacy. Good corporate support will allow the data professional to address more complex business insights.
These approaches will potentially reduce bias and lead to better interpretations. When the technology matures, Berthold thinks companies will look back in wonder. He said:
“We will be amazed by the results that we get when some of the people use generative AI. Additionally, we will be amazed by some of the failed attempts and note doing those tasks is not as easy as they seemed.”
In the end, generative AI will make the Data Science profession more interesting, as humans use it to discover new insights.