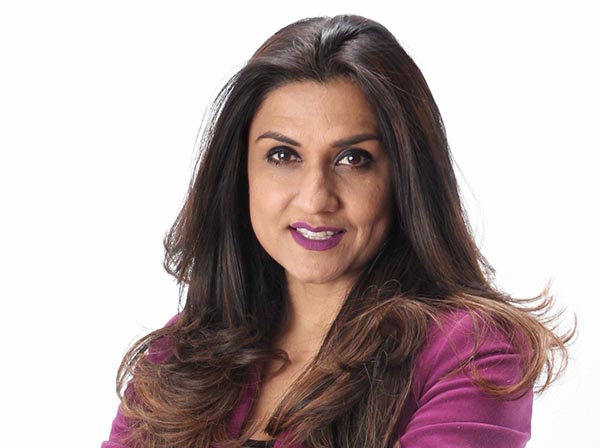
Analytics and big data are everywhere in the pharmaceutical industry providing insights into marketing, sales, clinical trials, claims data, patient demographics, physician engagement, and much more. However, before delving into deep analytics, you must master the data, or the insights that you get will at best, be inaccurate, but at worst, cause major issues within the enterprise. Healthcare lags other industries when it comes to data sharing and interoperability. Ignoring the role of Data Governance in healthcare is often penny-wise but pound-foolish.
According to research from Stanford Medicine Health trends report in 2018, the obstacles to data sharing in health care are numerous and include:
- Accessibility: Consumers and health care providers are reluctant to share data with tech companies and non-traditional health care sectors.
- Data Quality: Most data requires thorough cleaning and structure alignment in order to be referenced and shared between systems; there are not enough processes to ensure cleaning gets done.
- Physician Burnout: Doctors are becoming increasingly frustrated with inputting data into EHR systems and find themselves with less time with their patients.
- Privacy and Ethics: Patients are largely uncomfortable with their data being used for research and other purposes; the industry lacks any explicit permissions or anonymization processes.
What Is Data Governance, and How Is it Used in Healthcare?
Data Governance is a process of managing data over time to ensure that they meet organizational quality standards. Users need to trust their data, and this is the role of Data Governance, but in a comprehensive Data Governance program, the organization and its users are held accountable for creating high-quality data. They are also charged with using that data in an approved, secure, and ethical way. A unified Data Governance platform helps organizations to better understand their data – whether structured or unstructured – and properly govern it to mitigate compliance risks and empower information stakeholders.
The Data Governance teams oversee these processes, and ensures, for example, that data is accurate from multiple sources, which of these sources is the “trusted” source for the most accurate record and ensures that the data does not age – that is refreshed and updated with the most accurate information. For example, a pharmaceutical company might get different records from multiple sources about a given physician, Dr. Robert Smith. One vendor might list Dr. Smith at NYU, where he did his residency, while another vendor might list Dr. Smith at a practice in Connecticut, where he worked for a year. The user might go to this address and find that Dr. Smith left that practice and is now licensed and practicing in New York.
So, which one of these is correct?
Master Data
Master Data Management (MDM) is a method used to define and manage the critical data of an organization to provide, with data integration, a single record, or source of truth. The MDM tool takes into consideration disparate data sources and reference data and applies rules to determine the best record, which is then refreshed into all other systems in the organization, from CRM to SAP, to incentive compensation.
This is the concept of a “Golden Record” where the Data Governance team puts the following rules in place to solve the simple example above.
- If there are multiple addresses for a physician, determine the most recent address.
- If the most recent address is in a different state, determine if the physician has multiple state license records.
- If the user states that they are at another practice, allow them to update the record, pending a Data Stewardship check.
What Is Data Stewardship? A Case Study
Health Catalyst defines data stewardship as “the aspect of health care Data Governance that focuses on providing the appropriate access to users, helping users to understand the data, and taking ownership of Data Quality. When there’s poor Data Stewardship, however, even the best infrastructures become underutilized and poorly understood by knowledge workers who could be generating value from the data every day.
Data Stewardship was implemented at a pharmaceutical company where there were hundreds of thousands of records that were “unmatched” – where the users had free reign to edit and enter records with no regulation. The records that were purchased and put through the master data management process in this example, were then “polluted” by inaccurate data. Users, for instance, would rename doctors so that they would know which was “their doctor,” or enter duplicate records rather than searching for a doctor that was already in the database. Eventually, it was hard to provide accurate incentive compensation or forecast sales. To complicate matters further, a new sales system was put in place that only allowed the sales reps to see a fraction of the doctors and hospitals that they were used to seeing.
At first, the system owners wanted to purchase data from a third-party source, make it impossible for the users to add or change data, and get rid of the Master Data Management system entirely. This was not feasible for five reasons:
- No purchased data was entirely accurate. Often, two different data sources provided information on multiple doctors’ offices.
- There was a 3-6 month lag in updating records for physician moves.
- Practices and hospital systems were being merged and bought at a high-enough pace that the purchased records were not up to date.
- While the user entered data had risks for data quality, the sales reps had a reason to enter accurate data – it ensured their proper compensation.
- Because the sales reps were only seeing a fraction of the doctors and hospitals on their iPads they would be unaware of records that were available for their use, but housed on the server rather than on their iPad.
Instead, we recommended that the company implement a Data Stewardship request program for the update of records by sales reps. A team of data stewards was hired to monitor and update data for records that were unmatched by the system as well as requests from the sales reps:
- The sales representative would enter a “request” for a new record or to change a record, with the new Name, Address, or Practice Name.
- In order to enter a new record, the sales representative would have to search online to see if the record already existed on the server. If the record did exist, they could request that the doctor or hospital be synched down to their iPad without any intervention from the data steward.
- If there was a change or a new record, the data steward would review the request, verify it with multiple sources including calling the practice, and then approve or reject the request.
- The updated record (if approved) would be synced down to the Reps’ iPad.
This process increased data accuracy to 99% taking into consideration user feedback, Data Quality, and an approval process that reduced mistakes, misspellings, or duplicate records. This clean data could then be used in incentive compensation, for forecasting, for analytics, with much higher accuracy.
Building a Data Governance Function in Healthcare
If you are struggling to understand and implement a Data Governance function in your healthcare organization, it is important to build a lean and balanced Data Governance function that will help your healthcare organization maximize the value of your data to deliver the best possible information to improve sales outcomes and reduce inefficiency. You can subscribe and listen to my Dive With Data podcast interview with Isaac Wagnor, Director of the Strategic Analytics group at Memorial Sloan Kettering Cancer Center in New York about “The Multifaceted Role of Analytics Governance.”