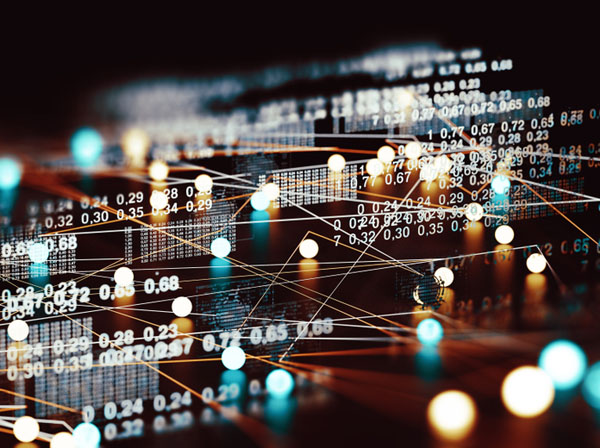
Data Quality tools can help to make data more trustworthy and more manageable. Inaccurate data promotes poor decision-making, missed opportunities, and lower profits. As use of the cloud continues to grow and become more complex, Data Quality has become a critical issue. Data Quality tools, when used effectively, resolve the issues that cause these problems.
A business’ data can be an invaluable resource. Errors and anomalies within the data are found and corrected by Data Quality tools, using algorithms and lookup tables. They remove typos, formatting errors, redundancies, and other issues.
Over time, these tools have steadily improved, becoming more automatic, more sophisticated, and easier to use. They can handle a variety of tasks, including:
- Validating mailing addresses and contact information
- Data consolidation
- Data mapping
- Sample testing
- Data validation reconciliation
- Processing big data handling
- Data analytics
Different Data Quality Tools for Different Tasks
There are several different methods for managing and improving the quality of data. Several types of Data Quality tools have been designed to deal with specific issues, ranging from data cleaning to data transformation.
Achieving the goal of Data Quality can be considered a multi-step process. Although “perfect” data may not be achievable, using the appropriate tools in a modern data stack can significantly boost an organization’s Data Quality. It should be noted the variety of Data Quality tools continues to grow and should receive fresh research as tools are needed.
Data Cleansing
Dirty data supports inaccurate results and poor decision-making. Data cleansing prepares data for use by removing or correcting data that is incorrect, improperly formatted, incomplete, or duplicated.
There are several methods for data cleansing depending on how it is stored along with the answers being sought. The goal of cleaning data is to produce standardized, uniform datasets that can be used for research, business intelligence, and data analytics.
Data cleansing focuses on gaining the best Data Quality possible when making operational decisions and business decisions. Developing an emphasis on quality data as part of the organization’s culture will help in this process. When done correctly, staff will help to provide valuable insights for decision-making.
Additional benefits that come with data cleansing include:
- Increased productivity
- Streamlined business practices
- Better analytics
- Faster sales cycle
The ever-growing amounts of data most businesses experience require automation for the data cleansing process. The right tool can manage a variety of issues automatically, before they become serious problems. A tool can ultimately help organizations become more efficient and profitable.
The data cleansing tools offered by different vendors emphasize different strengths. For example, OpenRefine is quite useful when dealing with messy data. It provides a good solution for working with free and open-source data. Trifacta Wrangle, on the other hand, emphasizes speed in the transformation process and focuses on analyzing data. And Drake is a simple, easy-to-use tool for text-based data, designed specifically for data workflow management.
Data Enrichment
This is described as merging an existing database containing first-party data with third-party data taken from an external source. By combining data taken from more than one source, businesses gain deeper insights into their customers’ preferences. The ethics of collecting, selling, and using third-party data has been questioned, with Europe and California passing laws to protect the privacy of individuals. Data enrichment tools should be carefully considered, with the understanding they could become useless if the U.S. federal government passes similar laws.
Crunchbase Enterprise is a popular data enrichment tool for startups and lists information on investors, funding rounds, corporate titles, etc. InsideView takes a different approach, focusing more on CRMs (customer relationship management) and can be integrated with many leading CRMs. Clearbit’s unique approach to data enrichment tools excludes other data providers, instead collecting and providing all the information themselves.
Real-Time Email Data Validation
Email verification is a process used to verify an email address’ validity and is a necessary step when performing email marketing. When writing an email address, mistakes can be made, resulting in an invalid email address. Emails sent to these invalid addresses get bounced and reduce the deliverability score during email marketing campaigns. Real-time verification typically uses an API to avoid incorrect email addresses.
ZeroBounce seeks out spam traps, spam-linked emails, and returned hard bounces “at high speeds.” Mailfloss is described as a “simple” email verification tool with the same basic features. MailerLite is known for having some of the best delivery rates within the industry.
Big Data
Big data consists of large volumes of data, often from multiple sources, which may be structured, unstructured, or mixed. The goal of big data research is to find insights that support good decision-making. Big data research tools may be used to cleanse and pre-process this mixed assortment of data, before being used for research. The term “big data” is becoming less popular these days though because using massive amounts of data is becoming commonplace, and technologies are much better at dealing with it now.
Zoho Analytics software collects data, and then indexes it, allowing it to be searched and sorted, and will automatically detect any abnormal data patterns. It can handle small, midsized, and large business enterprise data as well as that for public administrations and nonprofits. A single user license works for 1,000 to 4,999 and it can be deployed on premises through mobile devices, or even through the cloud. Splunk can collect data, and then index it, allowing it to be searched and sorted, and will automatically detect any abnormal data patterns. It should also be noted that there are a number of open-source big data research tools.
Enterprise Data Quality
These tools refer to a class of software designed to maintain and organize stored information, and mesh effectively with the different applications being used by the business. It is meant to ensure all data is complete, accurate, and up-to-date.
Enterprise Data Quality tools accomplish this through the use of procedural controls, which include:
- A data validation system to ensure accuracy upon entry
- Scheduled data audits that make sure existing data remains relevant
- Data profiling that identifies existing data that does not meet the system’s requirements
- The ability to detect and merge, or eliminate, duplicate entries
Oracle Enterprise Data Quality software provides master Data Management, Data Governance and cloud services, and integrates data with customer relationship management. Uniserv, a Canadian company, offers a Data Quality tool for large enterprises that is extremely flexible and scalable, and provides excellent training.
Data Transformation
The purpose of data transformation is to extract data from one or more sources and convert it into a format appropriate for the company’s system. The “now usable” data is then stored in the correct location until needed. Data Management and data integration tasks rely heavily on data transformation when working with data warehouses.
With an ever-growing number of applications, programs, and devices continuously producing large amounts of data, these tools come in quite handy. They automate the transformation process, eliminating the need to do it manually. The reality of big data makes data transformation tools a necessity for operating efficiently and effectively.
The Cleo Integration Cloud tool automatically accepts and transforms any type of B2B data, from any source. IBM DataStage provides a cloud-ready integration tool that will clean, modify, and transform data conveniently. Informatica’s data profiling solution, Data Explorer, scans data from any source to seek out anomalies and hidden relationships.
Data Profiling
These tools scan through data to find patterns, character sets, missing values, and other essential characteristics. Data profiling is the process of reviewing source data content and identifying details and data points that are potentially useful for data projects. The three basic types of data profiling are:
- Structure Discovery: This scans the data to assure it is consistent and formatted properly, including a check on mathematics within the data (sums, minimums, or maximums). Structure discovery can help to understand how well the data is structured—for instance, the percentage of phone numbers not having the correct amount of digits.
- Content Discovery: Individual data records are examined for errors. Content discovery will identify which specific rows within a table contain problems and find systemic issues occurring in the data (such as phone numbers lacking an area code).
- Relationship Discovery: Seeks out and recognizes interrelated data. Source data is reviewed, with the goal of understanding its structure, its content and interrelationships, and identifying the potential for data projects. The process begins with a metadata analysis to find key relationships, and reduces the connections between certain fields, especially when the data overlaps.
Selecting the Right Data Quality Tools
Duplicate data, missing data, and incorrect data can undermine projects and decisions significantly. This is why finding the Data Quality tools that meet your organization’s specific needs can have a very positive impact.
Choosing Data Quality tools may seem intimidating, but careful research will provide the best solutions. It is worth making the time to do the research and select the most appropriate tools. Some of the questions that should be considered when choosing tools are:
- Needs: What are the business’ Data Quality needs?
- Price: Is the tool subscription-based or a one-time fee? Are there add-ons that will cause the price to inflate?
- Usability: Is it user-friendly? Will it accomplish all the desired tasks?
- Support: How much will be needed? The availability of live support from the tool provider may be an important factor in decision-making.
- Business Size: What is the size of the business?
Micro-businesses (10 employees or fewer) do not normally need much in the way of data cleaning tools. Small (10–50) and medium size (50–250) businesses begin to need these tools on a part time basis. Large organizations will generally need a team focused on ensuring Data Quality. Good tools can simplify their jobs, allowing them to focus on other quality-related tasks.
Image used under license from Shutterstock.com