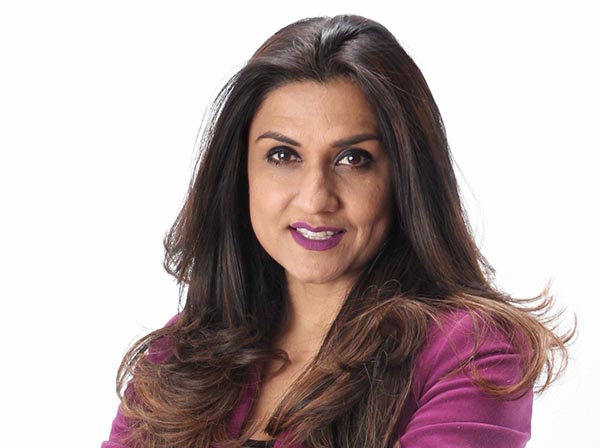
Click to learn more about author Asha Saxena.
The media buzz that surrounds Big Data, artificial intelligence (AI) and machine learning (ML) has never been higher, so much so that it can overshadow the real applications and actual outcomes companies are working on. But larger than life promises or hype might have an eclipsing affect around the actual, realistic benefits it provides to almost any organization, in a wide variety of industries, that are generating a large volume of data.
The use case benefits are real and it’s time for your company to start harnessing them. But before your organization can receive the value from AL and ML, it must get a thorough understanding of the role it can play at your business, the problems it can solve, and how it can align to your company’s objectives or intended outcome. The problem is that this is where a lot of AI and ML proof of concept (POC) initiatives have stalled and not made a lot of headways. To overcome this, companies need a place to start from. Smaller companies can take a cue card from tech giants such as Google which has started to make bets in solving large scale healthcare issues.
Large Tech Companies are Investing in Healthcare AI
Google AI, which has been doing AI related research and collaborative projects in the field of healthcare and biosciences says:
“Machine learning has dozens of possible application areas, but healthcare stands out as a remarkable opportunity to benefit people — and working closely with clinicians and medical providers, we’re developing tools that we hope will dramatically improve the availability and accuracy of medical services.”
An according article titled AI in Healthcare – A Key to Industry Evolution written by Ron Moody, who is Accenture’s chief medical officer:
“Artificial intelligence (AI) is poised to revolutionize healthcare operations, health research, the delivery of medical care and how patients are supported to maintain health. It is and will be a critical part of long-term healthcare solutions.”
AI is already infused into a variety of industry processes, apps and systems that people have daily interactions with, which is making the field of healthcare primed for an AI expansion. It will go beyond just using AI to support activities such a detecting diseases and medical diagnoses. This is just the tip of the iceberg.
The bigger picture of healthcare is similar to other commercial industries, administration, logistics, business processes and customer relations. These areas are where use case applications of AI have already started to make improvements to costs and efficiencies. Because healthcare costs remain under tremendous pressure to get lowered or brought down. These are some areas are that organizations should be exploring and investing into today to start making changes with using AI.
According to an article by Caserta “The United States spends over $10,000 per capita, or 18% of its GDP, on healthcare.” According to data from BGV, Global healthcare spending is projected to increase at an annual rate of 4.1% from 2017 to 2021, driven by an aging population, developing market expansion, and rising labor costs. Beyond digital disruption there has been unique opportunity for innovative startups to emerge and build technologies that tackle certain issues with the healthcare ecosystem.
The Value of AI
So, while already some healthcare organizations are already seeing benefits from applying AI to its operations, logistics, admin processes and even to improving customer engagement, AI also is showing promise augmenting areas such as pathology and radiology interpretation. With the increasing velocity of data and the fact the number of patient data sources will continue to expand, AI can be used in support of data processing, visualization as well as decision making support.
The application of AI through (ML) and natural language processing (NLP) can bring a tremendous amount of value across the current healthcare continuum to the delivery of improved outcomes. The use of these technologies in healthcare will also help support new models of “value-based care” and with the increase of Big Data – it can be leveraged to drive more personalization and transformation in healthcare to patients. Growth in the AI health market is expected to reach $6.6 billion by 2021—that’s a compound annual growth rate of 40 percent (see Figure 1).
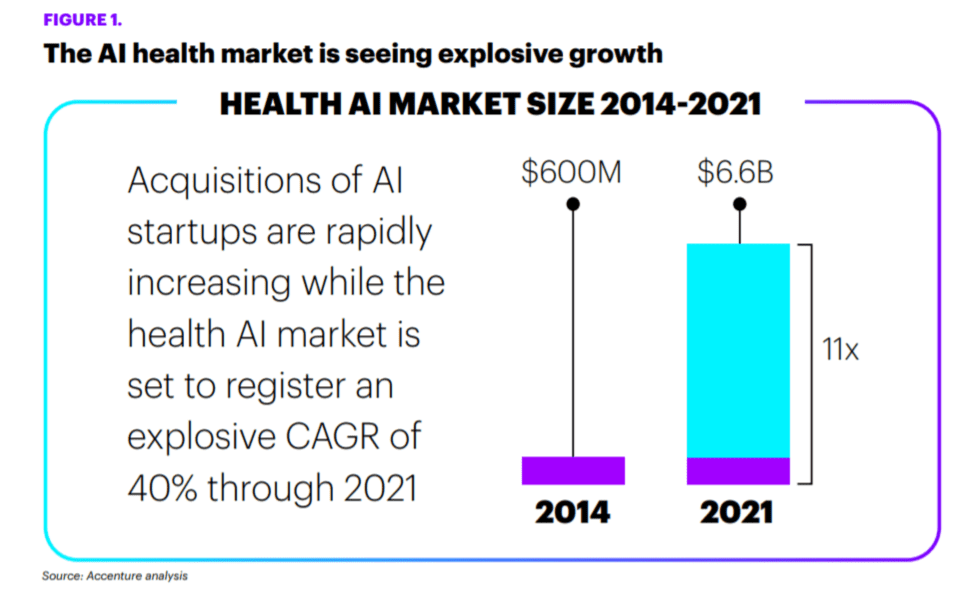
Deep Learning and Computer Vision Use Cases
The field of computer vision has already seen radical advances through an (AI) technique which is known as deep learning, or sometimes referred to as deep neural networks. Which is making useful in your pocket technologies out of could have seen like science fiction a decade ago.
If these new and advanced computer vision systems can have the accuracy of classifying different cars or breeds of dogs in images, Google’s engineers and scientists asked themselves “might those same systems be capable of learning to identify disease in medical images?” in a Google AI blog post Deep Learning for Detection of Diabetic Eye Disease Google’s Lily Peng MD PhD, Product Manager and Varun Gulshan PhD, Research Engineer describe that In the area of ophthalmology, how they began exploring computer-aided diagnostic screening for a disease of the eye called diabetic retinopathy. Diabetic retinopathy is the fastest growing cause of preventable blindness globally. Normally the test conducted by highly trained doctors examining a retinal scan of patient’s eye.
In the field of digital pathology, Google has been researching and developing deep learning algorithms that might assist pathologists in detecting breast cancer in lymph node biopsies.
Improving Diagnosis
In the last several years there has been a lot of progress made improving diagnostic accuracy of medicines using (AI) and (ML). And now according to research from Big Market Research the AI intelligence medical market is forecasted to exceed $18.12 billion by 2025. According to an article in AI for health, (AI) and (ML) are faster and more accurate in detecting anomalies in scans compared to humans.
By using (AI) and (ML) programs to detect anomalies that could be missed by a human eye are improving diagnoses and setting up patients to get better patient care. Here are few examples:
- Researchers at Stanford have created an algorithm to interpret chest x-rays that is just as accurate as radiologists are and can interpret results in a fraction of the time.
- Doctors using Viz.ai shave crucial hours off diagnosis time by using the technology to quickly and accurately detect blood clots in stroke patients before major damage can be done.
Lowering costs
In the U.S., more than 25% of health care expenditures are due to administrative costs, far surpassing all other developed nations. One important area where AI could have a sizeable impact is medical coding and billing, where AI can develop automated approaches.
According to data from an Insights at Forbes article AI And Healthcare: A Giant Opportunity the key to detangling the current healthcare system’s cost structure issue lies in the transfer of time-consuming human tasks to machines. While enabling patients to self-service their care needs wherever possible. This can help with reducing the amount of human labor that is required to keep more people living healthier lives. According to report form Accenture, when combined, key clinical health AI applications can potentially create $150 billion in annual savings for the United States healthcare economy by 2026.
Below is a chart of the top 10 healthcare AI projects that combined add up to that $150 billion savings number. Accenture’s assessment defined the impact of each application, likelihood of adoption and value to the health economy. The top three applications that represent the greatest near-term value are: robot-assisted surgery ($40 billion), virtual nursing assistants ($20 billion) and administrative workflow assistance ($18 billion).
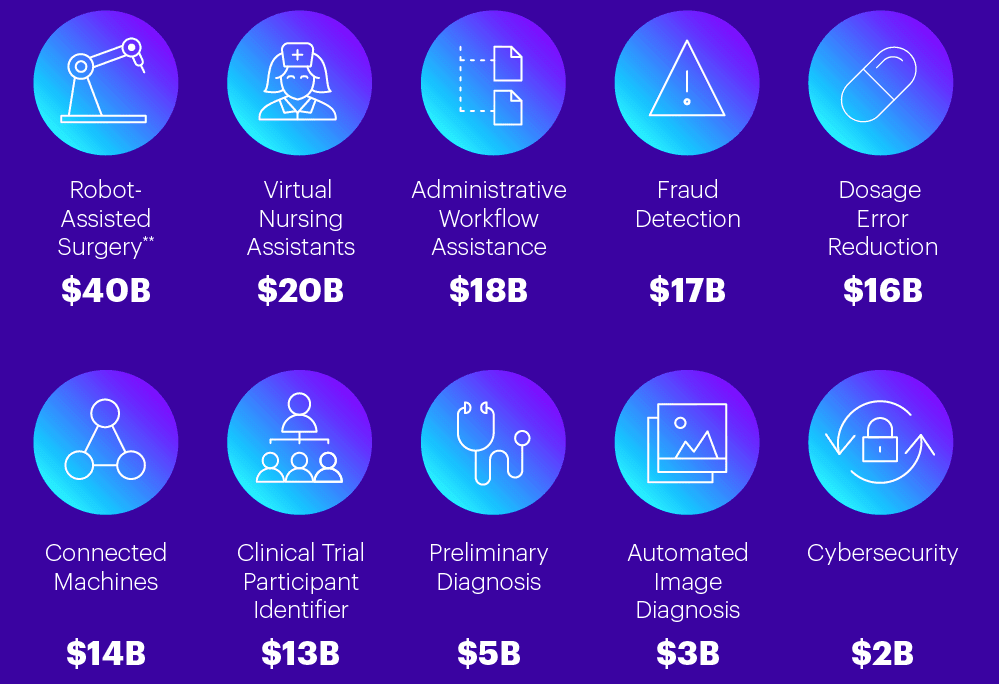
Detecting Healthcare Fraud
According to research from a Splunk report title AI and ML in regulated industries detecting and discovering patterns of anomalous and concerning behavior among a gigantic number of healthcare providers is difficult and very time consuming (sometimes taking months or years to complete). Locating the source of illegal prescriptions and monitoring it more closely is an additional problem or challenge. There has been all kinds of healthcare fraud abuses and scams, such as the prescription and distribution of opioids, which is the source of one of the nation’s deadliest substance abuse epidemics. (ML) can help discover anomalous and potentially fraudulent providers, that would be difficult and time consuming for humans to sort through and detect.
By aggregating claims data, it’s possible to get a full view into opioid purchases. With machine learning programs and algorithms, healthcare companies can see patterns across that data and when they deviate. This allows healthcare organizations to shift to preventing and detecting fraud, versus a “pay and chase” approach. According to an article from PBS News the Justice Department said, “Alleged fraud and false billings collectively accounted for 13 million illegal opioid dosages in the US” and also included 23 pharmacists and 19 nurses. The types of data sources can include; Electronic health records (EHR), health level 7 standard messaging, medical devices, desktops, servers, storage, network, portals, billing systems, patient management systems.
New Drug Discovery
According to a Medium article by Daphne Koller a ML researcher and startup founder of Insitro, big pharma has been struggling with developing new therapeutics. Over the past decades, developing drugs has become increasingly more difficult and expensive, leaving many patients with significant unmet need. Clinical trial success rates hover around the mid-single-digit range; the pre-tax R&D cost to develop a new drug (once failures are incorporated) is estimated to be greater than $2.5B; from when it was $200 million 30 years ago, and the rate of return on drug development investment has been decreasing linearly year by year, and some analyses estimate that it will hit 0% before 2020.
Regulatory oversight and not having large enough patient data sets has played a role the increased costs. Koller’s company is trying to change that, Insitro is trying revolutionize pharmaceutical R&D by leveraging machine learning for drug discovery and therapies. Insitro, in a matter of months raised over $100 million from big-name investors including ARCH, Foresite Capital, Andreessen Horowitz, and the firm that manages Jeff Bezos’ personal VC investments. On April 16th, 2019, the giant drug maker Gilead Sciences said it would pay Insitro $15 million — and up to $1 billion more down the line if it meets certain goals — for help in developing drugs to treat a common liver disease called nonalcoholic steatohepatitis (NASH), that is fast becoming a global epidemic fueled by poor diet and lack of exercise. The partnership deal calls for Insitro to create disease models to find the right targets to reverse the illness and offers a test of whether artificial intelligence can remake drug development.
Targeting Care
The first human genome sequencing under the Human Genome Project (HGP) took nearly 13 years and costed 2.7 billion. Since then the time and costs associated with sequencing individual genomes has gone down dramatically due advances in technology. Healthcare organizational providers are starting to use genome sequencing along with using patient data to optimize the care for an individual by tailoring it from the individual’s unique genetic profile. The new advances and development in precision medicine has been unlocked not only from genome sequencing but also with the explosion of using Big Data and cloud in (ML) techniques.
Machine learning algorithms can identify patterns and make predictions using cloud computing data lakes and data warehouses that clean (creating a single ‘source of truth’ in the data) and store huge amounts of data which enables the integration of multiple health care systems together. For the purpose of providing better and more targeted care to an individual’s electronic health record.
Oncology and cancer research have gotten the most investment into precision medicine by studying cancer genetics. In some instances, cancer treatments can be suggested based on genetic drivers of the cancer and not on the physical location of the cancer itself within the patient’s body. Moffitt Cancer Center in Tampa, FL has been working on incorporating molecular genomics, demographics and trial outcomes to develop models for each patient. The use case.
Of precision medicine will not only lower the risks of using sometimes incompatible treatments and medicines but it also building a new solutions for fighting disease and delivering healthcare. A startup called Deep Genomics uses (AI) and the genome to determine the best drug therapies for each individual.
As more healthcare organizations start to invest, experiment and integrate emerging technologies into their systems, traditional healthcare models will be disrupted and changed.
Conclusion
While there is plenty of use cases and evidence of the promising benefits of using (AL) and (ML) applications in the healthcare industry, and each year more VC funding continues to flow into the sector, there are still a bunch of challenges for healthcare and tech startups beyond the mere improvement of technology to address a large potential market. Some of these challenges include:
- Getting widespread adoption
- Implementation of these tools
- The healthcare ecosystem
- Regulations
- Current business models
- Incentive alignments with the Payer-payee relationships
Healthcare organizations have to learn to trust algorithms to use them, meaning they using want to see clinical validations of them. As of now some are still cautious or hesitant about going into a full force adoption of (AI) tools without having a large body of proof that verifies the outcomes.
It’s not just the doctors who are skeptical, according to a digital health survey conducted by Accenture, around 25% of the respondents are not ready to use (AI) powered health services. With many citing they had some concerns about the technology, ranging from simply not understanding how (AI) works or that they fear the technology will truly understand them.
Another challenge to widespread adoption of (AI) in healthcare is scalability problems. The proof of concept (POC) or pilot programs that are often tested with a limited scope may not be quite ready to be rolled out or adapted to large scale institutions. Another issue is that advanced (AI) technology solutions at the moment might be a little too costly for the smaller regional and rural healthcare facility organizations, or at least initially.
Finally given the huge scale of, and diverse range of stakeholders involved in, the current health industry, anticipate some push and pull as (AI) disrupts and revolutionizes healthcare operations and treatments for healthcare executives, clinical practitioners and patients alike. The future is looking promising, Morgan Stanley estimates that the global market for AI in healthcare could surge from $1.3 billion today to $10 billion by 2024, growing at an annual compound rate of 40%. You can subscribe and listen to my Dive With Data podcast interview with Vijay Venkatesan, Chief Analytics Officer of Horizon Blue Cross Blue Shield of New Jersey about “How Data Analytics Can Be Used in the Healthcare Industry to Improve Patient Care.”