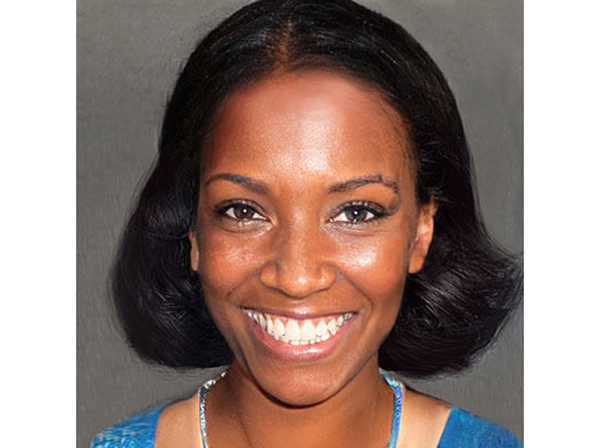
Data-centric AI (DCAI) is a new class of AI technology that focuses on understanding, utilizing, and making decisions based on data. Before data-centric AI, AI was largely reliant on rules and heuristics. While these could be useful in some cases, they often led to suboptimal results or even errors when applied to new data sets.
Data-centric AI changes this by incorporating techniques from machine learning and big data analytics, allowing it to learn from data instead of relying on algorithms. As a result, it can make better decisions and provide more accurate results. It also has the potential to be much more scalable than traditional AI approaches. In the future, data-centric AI will likely become increasingly important as data sets grow in size and complexity.
This article will dive into data-centric AI and explore its potential implications for businesses and society.
What Is Data-Centric AI?
As mentioned, this type of AI is designed to work with data to learn from it and make predictions. Businesses often use data-centric AI to make decisions about products, services, and marketing. For example, with VoIP phone services, data-centric AI can help understand customer usage data and decide how to improve the service.
Data-centric AI is often used together with other types of AI, such as machine learning and deep learning. It is becoming increasingly popular as more businesses and organizations realize the potential benefits of using data to make decisions.
How Does Data-Centric AI Work?
Data-centric AI can help improve the performance of AI services with augmentation, extrapolation, and interpolation. By increasing the amount of data available to an AI service and making it possible to use that data more effectively, data-centric AI can help make those services more accurate and reliable.
With this new approach, data-centric AI is generated using training data from different sources, including synthetic data and public and private data sets. This approach can help improve the quality of training data and reduce the amount of time and effort required to generate it. In addition, it can also help improve the efficiency with which AI services use training data. And because the data is tailored, data-centric AI will almost certainly be able to process additional data sets as well.
This means that no matter how big or small the data set is, data-centric AI can learn from it and make predictions. Additionally, data-centric AI is not limited to a specific type of data. It can learn from text, images, audio, and video.
In general, a data-centric AI strategy comprises the following steps:
- Using the appropriate labels and fixing any problems
- Getting rid of the noisy data instances
- Data augmentation
- Feature engineering
- Analysis of error
- Employing domain experts to identify the accuracy or inaccuracy in data points
Comparing Data-Centric AI to AI-Centric Data Science
There are a few key differences when comparing data-centric AI to AI-centric data science.
First, data-centric AI is focused on using data to drive the development of AI models. The data is used to inform the training, testing, and evaluation of AI models. In contrast, AI-centric data science focuses on how AI models use data for learning and decision-making. It can include things like preprocessing data, feature engineering, and model selection.
In addition, data-centric Al can also help smoothen AIOPs (AI model operationalization process) by providing data that is happily formatted and cleaned for use. Cleansing data can be time-consuming, but it is important to the success of an AI model.
Optimizing and Operationalizing AIOps Functions
As businesses strive to operationalize their AIOps functions fully, data-centric AI has emerged as a critical piece of the puzzle. With data-centric AI, it’s all about using data to learn and improve upon algorithms and processes. In other words, data is used to “train” the AI system so that it can make better decisions and take actions that will optimize and improve AIOps functions.
There are several reasons why data-centric AI is so important for AIOps:
- Machine learning models require a lot of data to be effective; the more data fed into the system, the better it will be at making predictions and taking actions. Storage technologies and cloud computing have made it possible to store and process large amounts of data helping to train machine learning models on an unprecedented scale.
- AIOps functions are constantly changing and evolving. As new data is generated, it’s important to be able to incorporate that data into the AIOps system so that it can improve its performance. Data-centric AI allows for this constant learning and evolution so that the AIOps system can always be as effective as possible.
- Data-centric AI helps to identify patterns and relationships that would otherwise be difficult to discern by analyzing large amounts of data. This information can then be used to improve the AIOps system to identify and solve problems more effectively.
- Data-centric AI can be used to identify outliers and anomalies as well as unusual patterns of behavior. This information can then be used to investigate further and take corrective action if necessary.
- Data-centric AI can help to automate AIOps functions. And automating the process of collecting and analyzing data can help improve the efficiency of AIOps functions. This can free up time and resources so that they can be devoted to other tasks.
Data-centric AI is a vital piece of the AIOps puzzle. Without data, AIOps functions would be severely limited in their ability to operate effectively. This type of AI can have uses in health care, finance, crypto wallets, digital currencies, and many other industries.
The Future of AI
AI is evolving rapidly, and data-centric AI is emerging as one of the most promising new approaches. With the intention to process and make decisions based on data, data-centric AI has the potential to enable more intelligent and efficient systems.
In a world where data is becoming increasingly important, data-centric AI has the potential to revolutionize how we interact with information. By understanding and utilizing data in new ways, it can help us make better decisions, automate tasks, and even improve our understanding of the world around us.