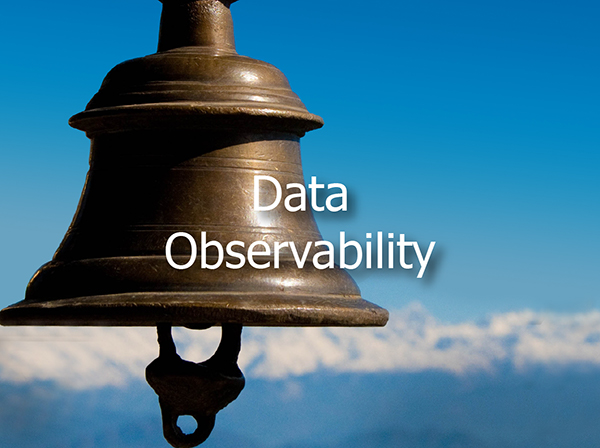
Data observability is the practice of monitoring and analyzing the health of an organization’s data and data systems. Essentially, it gives you a 360o overview of what’s happening with your data at any given point in time. This practice is beneficial, as it provides all stakeholders with an in-depth insight into how their data is collected, stored, used, and processed. Moreover, it enables them to understand whether their data is reliable and can be used to fulfill overarching business objectives.
While the terms “data observability” and “data monitoring” are often used synonymously, there’s a key distinction between them. The former goes beyond simply monitoring the data, as it also regularly evaluates the applications, tools, and servers it’s being processed through – and assesses the health of those systems too. That means that you keep track of its metrics (data measured over time), analyze its logs (timestamps of events), and determine its traces (causally related events). Ultimately, your data is only as good as the system processing and analyzing it, so evaluating the backend is crucial.