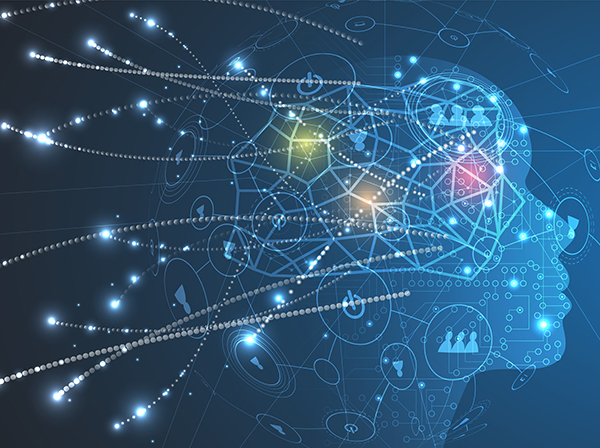
Every day, trillions of data points are generated, and, in theory, organizations can harness this data to make better, more informed decisions. But it’s precisely the vast scale of available data that makes it so hard to manage. According to IDC, data professionals spend 80% of their time searching for and preparing data and only 20% on analytics. This is why business leaders are increasingly turning toward automation and AI technology to quickly locate data, assess its integrity and relevance, and achieve data intelligence.
What Is Data Intelligence?
Data intelligence refers to the capability of turning all that raw, unanalyzed data into easy-to-digest information. “Data Intelligence is the engine of automation behind managing your data, making sure that your data is well equipped, fit for purpose, and ready to use,” said Susan Laine of Quest Software in a recent interview with DATAVERSITY. “The industry has really gone full throttle into AI, automation, and data lineage so that we can better tell the story of data.”
Laine herself is no stranger to “the story of data,” having played a key role in the emerging field of data intelligence. As the director of solution specialists and global pre-sales team at Quest, she has witnessed the data intelligence revolution unfold, placing her in a prime position to weigh in on its uses, benefits, and trends.
From Raw Data to Actionable Insights
As a specialist in her field, Laine has spent years observing clients struggling to understand the scope of their data and get value from it.
“If you just get a pile of data, that’s all you get – a pile of data. There’s no meaning, nothing behind it,” she said. “People need quick ways of getting to the context of the data. What they’re looking for is a map of exactly where their data is, how that data is moving, and how that data is described and being used.”
Data intelligence tools can provide just that map, serving as an intermediary between a sea of data and the people who work with it. Businesses use data intelligence tools to:
- Automate time-consuming tasks such as updating spreadsheets and tagging metadata
- Monitor and improve data quality
- Trace data lineage to ensure trustworthy data
- Support and advance data governance and stewardship
- Locate sensitive data that may pose a risk to compliance
- Empower citizen data scientists (business users tasked with analyzing data)
- Improve the overall visibility of an organization’s data assets
- Enable collaboration between IT, data governance teams, and business users
While the specific practices of data intelligence may vary from organization to organization, the goal is the same: to ensure everyone in the business can trust and use their data for meaningful insights.
“End users can’t use data on a day-to-day basis if they can’t find it and don’t trust it,” explained Laine. “So, having data intelligence and immediately finding and understanding the data – for example, validating that this new AI program I have is using reliable, validated data – will make the outcomes we’re trying to achieve much more intelligent.”
Why Organizations Need Data Intelligence
A critical part of any digital transformation strategy, data intelligence empowers businesses to discover and use data assets that might otherwise be invisible or at least camouflaged. For instance, automation-powered data intelligence tools can reveal unidentified third-party data, effectively “dark matter” databases whose existence was unknown to management. The result is an acceleration of data use and a reduction in the data “bloat” so common today, said Laine:
“The key is to be able to look across the different lines of business to not just manage the data for one particular area, but to be able to really understand quickly the source of that information and any sort of root cause analysis that undoubtably impacted many business units.”
Identifying critical data and everything associated with that data can be one of the biggest roadblocks to effective data utilization. Clients using traditional, manual means of vetting critical data often delay data intelligence program deliveries with vendors simply because the process of vetting critical data – finding it, fixing it, performing impact analysis – can create such time lags that by the time the data elements are presentable, the realities of the business have evolved so radically that the data is no longer relevant.
“Once people start to dig in and do the forensics around that data, they realize that they can’t do it manually – there’s just too much data,” said Laine. “Meanwhile, a data intelligence tool can get in there and scan those same applications and start making connections between the “head” and the “body,” connecting business assets to technical assets, so you can start to see the full story of what’s going on with the data.”
Putting Data Intelligence into Action
The success of data intelligence tools comes down to the customer experience of the data analysts and citizen data scientists who use them daily. As Laine sees it, the “intelligence” of data intelligence lies in its ability to improve business operations in a way that is “built into the recipe, if you will, or the ingredients of what you’re baking. It’s about speeding things up to the point where you can actually use that data to go out and disrupt the marketplace.”
It was in the service of such disruption that Laine arrived at Quest to work with the erwin by Quest organization this year. erwin was acquired by Quest in 2021 and is known best for its popular data modeling tool and newer integrated data intelligence suite combining data catalog, data literacy, data quality, and automation features.
“When erwin came out with their automated data intelligence offering, that’s what really intrigued me – the way it mapped the critical data elements, the tagging, the taxonomies, the logical information,” said Laine. “With a click of a button, I could see the physical lineage and turn it into logical lineage and read the names of those columns across the lineage in an English business manner.”
The latest version of erwin’s data intelligence platform features integrated and automated data quality capabilities, as well as a new consumer-like asset discovery and collaboration experience for non-technical business users. Dynamic search capabilities in tandem with immediate access to associated data literacy aids such as data lineage, data quality information, and data relationship mind maps speed the ability of users across the organization to find, assess, and understand how best to use the data assets available to them. Conversational chat, to-do lists, and socialization capabilities also improve engagement and collaboration between business users and data governance teams to raise data knowledge for all.
It’s all in the name of making data as accessible and understandable as possible so that everyone in the business can – and will – take full advantage of it. “We have to make it fun and familiar for the end user when they’re working in that space,” stressed Laine. “The more that we can automate the curation, the tagging, the categorization, the taxonomy of the data, the better we can help the end users make those decisions and have stronger insights.”
About Quest
Quest creates software solutions that make the benefits of new technology real in an increasingly complex IT landscape. From data intelligence and data modeling to database and systems management, platform management, and cyber security resilience, Quest helps customers solve their next IT challenge now. Learn more about erwin by Quest at erwin.com.
Image used under license from Shutterstock.com