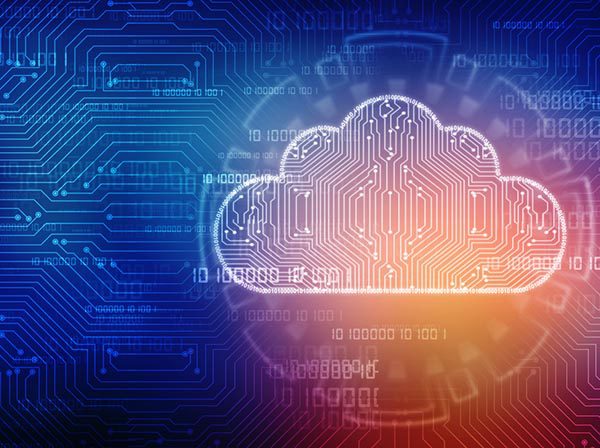
The axiom goes that the difference between high-performing enterprises and lower-performing businesses is that the former maximizes their data and the latter doesn’t. But most businesses probably still live somewhere between those two worlds.
That means a lot of companies are still working hard to get to the next level for IT-supported business initiatives including increasing operational and/or workforce efficiency, improving customer experiences, reducing costs, and improving access to data for decision-making.
What are the means that could help them get there? The top two technologies that have provided business benefits so far are infrastructure modernization and cloud/hybrid computing, said Harald Smith, the Director of Product Management at Syncsort, in a recent DATAVERSITY® interview.
A recent survey by Syncsort finds, for example, that 48 percent of the respondents said their organization was only somewhat effective in getting value from data. The percentage of respondents who believe their company is effective in getting data insight to users was split, with 50 percent of respondents believing their company is effective or somewhat effective doing so. But 35 percent are still neutral on the matter, and 15 percent continue struggling.
Advanced/predictive analytics and Data Governance, with each one hovering near the 30 percent mark, were next in line. Both technologies can have strong implications for a company’s desire to improve their customer experiences, helping them gain a holistic view of how their customers’ preferences, buying habits, and other data points can be discovered and used for sales, marketing, and other tasks.
Staying the course of what has given them value to date, respondents say their highest priority over the next twelve months is cloud/hybrid computing, infrastructure modernization, and data governance.
New Infrastructure for Next-Generation Analytics
As much as there are benefits, there understandably can be issues to address when it comes to moving data and redeploying processes from legacy platforms to Big Data platforms and the cloud to reduce costs and achieve higher processing performance.
“There is a desire to modernize infrastructure into Big Data platforms or clouds,” commented Smith. Syncsort provides solutions for data infrastructure optimization, the cloud, data availability, security, data integration, and data quality. As businesses build data lakes to centralize data for advanced analytics, they need to also ingest mainframe data for Big Data platforms like Hadoop and Spark.
According to Smith, the top analytics use cases that drive data lakes and enterprise data hubs are advanced/predictive analytics, real-time analytics, operational analytics, data discovery and visualization, and machine learning and AI. The top legacy data sources that fill the data lake are enterprise data warehouses, RDBMS, and mainframe/IBM I Systems.
“Legacy infrastructure is so critical,” Smith commented. “These systems are not going away.” Mainframes and IMB I still run the core transactional apps of most enterprises, according to the company. “Very traditional environments are central to how you order and deliver goods or how you keep your business running,” he said. So, as legacy processes move into Big Data or cloud platforms, it is just as important to have immediate failover.
Data moved from the mainframe into the data lake – and merged with other data – now is able to be analyzed at lower costs and at greater scale. But appropriately shuffling data across environments isn’t a one-time process, as the mainframe applications continue to update their data and the data in the data lake must constantly be kept in sync with that. Keeping data up to date and in sync is among the top IT challenges identified by Syncsort, as is user access to data.
To help cope with this, Syncsort’s Connect CDC product captures changes on the mainframe in real time as transactions are completed by reading directly from the mainframe database logs. In addition to updating the data, it also updates the Hive metadata with data location to keep analytics queries running fast. “There’s no need to have specialist coding,” Smith says – the workflow is defined in an intuitive GUI.
There has been a lot of uptake in Syncsort’s Change Data Capture for keeping data in the data lakes current, Smith noted. “It’s key to make sure the information you get and access out there is not stale,” he says.
Govern with Governance
Though Data Governance is one of the factors singled out for providing business benefits, Smith mentioned. “Data integration and data quality and security are all integrated with Data Governance,” he said, whether for compliance, risk mitigation, business value, or cost.
There are plenty of traditional data quality challenges within the business itself to overcome in order to provide good information to data scientists and business intelligence analysts from a central data lake. But those data quality issues – such as knowing the lineage, completeness, and accuracy of the information that comes from multiple sources – become even more of an issue when dealing with third-party data.
“I may have good confidence in how I assembled data from operational systems and how I brought that data in, but how do we know how a third-party gathers data?” Smith asked. Did they collect it in compliance with GDPR requirements? Is there an inherent bias within the data that could impact the ability to deliver valuable insight from analytics? If you’ve asked the supplier to document and profile the data, can you trust that they’ve done so in a way that is understood, repeatable, and accessible?
Additionally, some organizations have yet to determine how to operationalize and govern technologies still in the nascent stages of research such as blockchain, IoT and AI. These are the three top technologies under evaluation by companies. Without well-governed data, the ability to take advantage of machine learning and AI are at risk.
Syncsort’s efforts to address data quality issues pertaining to Data Governance and integration are reflected in its Trillium DQ for Big Data solution as well as its partnership with enterprise information management and IT systems management vendor ASG. Customers can be provided with data profiling and validation for business rules (created by the user to execute the technical implementation of data quality and policies) so that analysts in the lines of business can immediately identify issues and anomalies, according to the company.
Trillium data discovery and quality software with ASG’s Enterprise Data Intelligence makes it possible to track where data comes from and how it moves through systems by managing and defining business level policies, aligning business terms and quality results with key data, and gaining insights into where data quality gaps exist from originating sources to end destinations, such as BI reports. “You have lineage back to data,” Smith said. “That is critical in the data lake.”
Image used under license from Shutterstock.com