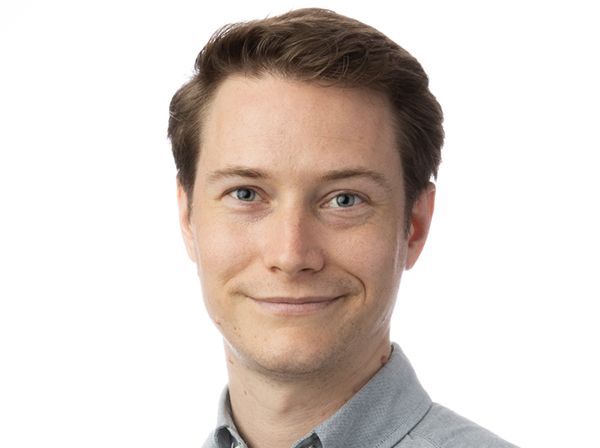
We’ve all generally heard that data quality issues can be catastrophic. But what does that look like for data teams, in terms of dollars and cents? And who is responsible for dealing with data quality issues? To get to the bottom of these questions and more, we conducted a survey of 100 survey respondents, at least 63 came from mid-to-large cloud data warehouse customers (with a spend of more than $500,000 per annum) who have some form of data monitoring in place, whether third-party or built in-house. Here are some important patterns we noticed.
Upstream Changes Are the Most Common Data Quality Issue
Thirty-one percent of respondents told us that upstream changes are the most common data quality issue they face. When schemas, data types, and formats change, that can impact all of the data downstream and pollute analytics. If upstream changes aren’t properly communicated to downstream data consumers, that’s when teams tend to see issues.
To address this problem, respondents recommended automation – for example, implementing Github automations that tag PRs involving data model changes with reviewers from the consuming team. They also recommended data SLAs – contracts that specify formal commitments to the data’s framework and quality, with penalties for violating the contract.
In Data Quality Work, Data Scientists Share the Stage
The research found that the “data engineering” role is now as popular as the “data scientist” role. “Data science” has repeatedly topped “hottest jobs” lists, but now those roles are joined by others. They are data engineers (in charge of managing data pipelines and data quality) and data analysts/business analysts (consuming the data, either by building dashboards or by using the data to drive business decisions).
Data-as-a-product is growing more prevalent on technical teams. That’s why new disciplines like data engineering aim to bring best practices from traditional software engineering (like observability or site reliability engineering) into the data product. Data quality work is officially becoming the purview of data engineers and software engineers, with smaller contributions from data analysts.
“Severe” Data Incidents Are Common
In our research, we defined “severe” data incidents as those that impact the company’s bottom line. Twenty percent of respondents reported at least two “severe” data incidents in the last six months, which created damage to the business/bottom line and were visible at the C-level. Data quality and reliability issues currently pose significant challenges for organizations, from customer impact to overall productivity.
Further, 70% of respondents reported at least two data incidents that diminished team productivity. That means that in a best-case scenario, most teams are inconvenienced by data incidents; for the unlucky 20%, data incidents cause major problems.
Software Engineers and Data Engineers Feel Disempowered
Survey results highlighted that both software engineers and data engineers feel disempowered when it comes to fixing data quality issues. What are the reasons? Lack of incentive across the team at large; a warrior of one has a difficult time winning a battle against a large-scale data issue. Additionally, respondents noted a lack of visibility into the root cause; how can you fix something you can’t understand? Lastly, both software engineers and data engineers reported a lack of ownership over the ability to fix data pipeline issues, due to role and command structure.
Third-Party Data Monitoring Over In-House Builds
Respondents who used third-party data monitoring solutions found approximately two to three times higher ROI over in-house solutions. By using a product whose core business is data quality monitoring, data teams found that they freed up more time to turn their attention to their core business functions. They also noted that third-party data monitoring solutions had better test libraries and a broader perspective on data problems. At full utilization, respondents noted that third-party monitoring solved for two additional issues: fractured infrastructure and anomalous data.
Final Thoughts
At the end of the day, automation, schema validation, source checks, and comprehensive monitoring are necessary for most data teams. Data quality is no longer an afterthought; in fact, the practice of data quality monitoring will likely grow more comprehensive and become standard as best practice across most industries that have a technology component.