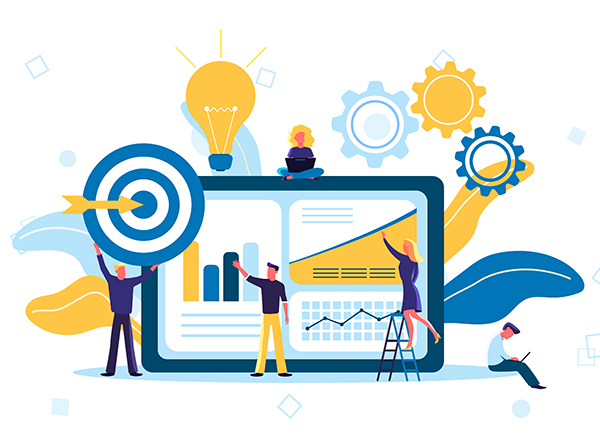
Data and analytics are particularly critical to today’s businesses because they improve strategic decision-making. Analytics solutions and use cases provide customers with added value in health care, retail, higher education, manufacturing, and other industries that capture a lot of valuable data.
By harnessing different types of analytics available, organizations across varying industries can understand how products are used, why specific outcomes happen, and even how to predict the future and get insights into how to alter future outcomes – completely changing how businesses operate. Some self-service business intelligence platforms offer the option of embedding analytics dashboards in applications, making it easier for people to analyze data.
Data Analytics Solutions in the Age of Data Science
The evolution of Data Science and the advancements of analytics have jointly led to an extensive array of applications, which are providing better insights and enterprise value. Whereas conventional analytics is a process that uses historical data to make better decisions about the future, predictive models try to predict the future. Predictive models are particularly helpful in real-time environments, where the data being analyzed is created in real time. With a number of predictive analytics techniques, as well as a thorough review of the historical data, it is possible to construct models for future events.
While predictive analytics has been around for decades, Data Science applies machine learning and other algorithmic approaches to large data sets to enhance decision-making capabilities, creating models that better predict customer behaviors, financial risks, market trends, and so forth. Analytics helps uncover insights and leverage them to enhance the decision-making processes and effectiveness of businesses. Analytics is the tool that helps in making data useful, allowing a better understanding of processes, and improving business productivity.
To explain how Data Science, AI, and ML have transformed enterprise business analytics in the current decade, this article presents three mini cases, with noted industry experts and market leaders weighing in.
One of the biggest uses for enterprise data analytics today is to forecast events – predicting, say, when a machine is going to break down, or how much inventory is needed in a given store at a given moment.
Analytics Solutions for Big Data: Applications and Use Cases
The availability of innovative solutions on the market has been a game-changer for organizations that could not use their massive amounts of data before. As the market and technologies have matured, sophisticated and cost-effective Internet of Things analytics solutions are turning data that was not accessible before into insights that are literally changing how companies do business.
Big data analytics is a process of reviewing huge volumes of business data sets from disparate sources to extract key insights, such as hidden patterns in customer data, correlations, ongoing market trends, customer preferences, behaviors, and so on. Big data enables analysis of data that is not being utilized in the production side of the business.
The primary goal of big data analytics is to provide actionable insights to business leaders to help them make better business decisions. Multiple industries are using different use cases for big data to drive enterprise success by analyzing vast amounts of unstructured data.
- In the sensor-driven Internet of Things (IoT), various types of advanced analytics solutions help in making sense of real-time data from IoT. Solutions such as ThingWorx help organizations turn their high-volume IoT data directly into actionable insights that can improve decisions made in various business functions.
- In insurance claims administration, big data-driven predictive analytics is used to enable faster services by analyzing massive amounts of data at the underwriting stage. Predictive analytics can be used to increase efficiency and mitigate risks.
- In the financial sector, big data analytics is used for risk analytics practices such as detecting money laundering, enterprise risk management, KYC, and fraud mitigation. In most cases, different types of financial institutions routinely apply insights from big data analytics to high-frequency trading, pre-trade activities, sentiment analysis, and more.
- The advent of deep learning, using artificial neural networks to analyze big data sets, has more effectively enabled organizations to perform analysis of unstructured data, ranging from image, object, and audio recognition tasks, to categorizing data based on the types of documents.
Top Solutions That Augment the Modern Data Analytics Process
Companies of all sizes are doing rudimentary analysis of operational data, but the increasing availability and sophistication of data analytics tools have expanded the types of insights businesses can collect. Modern data analytics platforms support embedded analytics, augmented analytics, and hands-free, self-service analytics.
Below, we’ve highlighted the top modern analytics solutions – the platforms and tools that have made all of the transformative analytics activities possible in any modern-day business.
- R and Python are still the best programming languages for the data analytics field. While R is an open-source solution for statistical analysis, Python offers easy syntax and dynamic semantics.
- Microsoft Excel, a favorite among a wide range of users from students to experienced professionals, provides sufficient features for extracting insights from data. Reportedly, it may not have the sophistication of a full-fledged analytics platform.
- Tableau is very popular for BI purposes, as it offers excellent analytics and visualization features. Named a “Leader” for Analytics and BI in the 2022 Gartner Magic Quadrant for the 10th consecutive year, Tableau allows users to work with live data sets.
- RapidMiner, named a “Visionary” in the 2021 Gartner Magic Quadrant for Data Science and Machine Learning Platforms, is exceptional for its model-building capabilities.
- Konstanz Information Miner or KNIME is an open-source, GUI-driven data analytics and reporting platform.
- Power BI, the second product from Microsoft featured on this list, was named a “Leader” in the 2022 Gartner Magic Quadrant for Analytics and BI. The notable features are self-serve BI, and advanced visualization tools to help users build dashboards and reports on their own.
- Apache Spark is an open-source analytics solution that supports “cluster-computing” and “real-time processing.” It also provides a fault-tolerant interface for programming.
- QlikView is noted for its data analysis, data visualization, and self-serve BI capabilities. Named a “Leader” in the 2022 Gartner Magic Quadrant for Analytics and BI for the 12th consecutive year, this solution platform includes niche features like data literacy and data integration.
- Talend was named a “Leader” in the 2022 Gartner’s Magic Quadrant for Data Integration Tools for the seventh year in a row; this solution offers step-by-step guidance for ETL process.
- Splunk was named a “Leader” in the 2021 Gartner Magic Quadrant for Security Information and Event Management (SIEM); this solution helps to search, analyze, and visualize data gathered from applications or websites.
Image used under license from Shutterstock.com