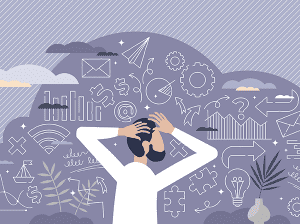
Leaders need to trust data within the organization to make sound business decisions. So, many turn to master data management (MDM), a solution to get and keep uniform and accurate data that increases business value.
Yet, according to Gartner, 75% of all MDM programs across organizations fail to meet business objectives. Moreover, this trend has worsened since 2015, a 9% increase.
“MDM is complex,” acknowledged Amy Cooper, principal consultant with Dun & Bradstreet and an expert working with clients wishing to evolve as data-driven organizations. “Aligning people, processes, and technology to govern and structure master data to get good Data Quality is particularly difficult,” she said.
To help businesses understand why MDM is so hard, Cooper shared her insights during a recent Data Governance & Information Quality Conference (DGIQ). She covered three MDM pitfalls and offered suggestions for addressing them.
Failing to Connect the MDM Program to Business Objectives
Cooper’s first significant pitfall is a failure to connect the MDM program to business objectives. She explained that many executives, the primary stakeholders, understand the need for MDM programs but lack knowledge about how they connect with business value.
Many executives do not even measure their MDM programs. She pointed out that 90% of organizations fail to collect key performance indicators (KPIs) for their MDM program.
“The 10% of companies do have metrics or KPIs tie it to the data itself, not the business outcome,” she said. Cooper illustrated her point using the diagram below.
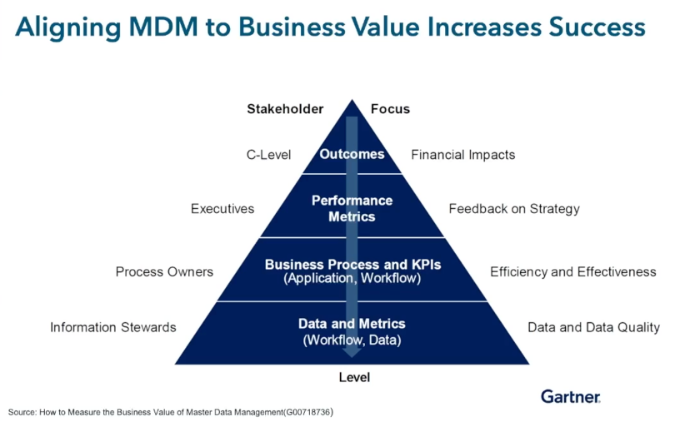
Figure 1 (Image Credit: Gartner)
She pointed to the left of the triangle at the C-Level, under “Stakeholder,” and said, “Executives tie the MDM’s program success with outcomes, such as growing revenue, reducing cost, mitigating risk, or operating more efficiently.”
However, tying metrics with the data and the workflow, as shown at the bottom of the diagram, leaves a gap. She noted this approach or not having any metrics makes it harder to advocate for MDM funding.
She explained, “Executives do not understand that MDM is ongoing. It is a mindset and a culture.” They are more likely to get this concept when MDM metrics connect with the outcomes that make up their focus.
Relying Solely on Technology
In addition to failing to connect MDM’s value with business outcomes, “People start with MDM by jumping in with the technology,” Cooper said. “Then, they try to fit the people, processes, and master data into their selected technology.”
Moreover, in the process of prioritizing technology first, organizations take for granted that they have good Data Quality, data that is clean and fit for purpose. Then, during a major initiative, such as migrating to a cloud environment, they discover their data is not so clean. They fail to budget time and resources to assess if Data Quality is good enough.
Engineers and their managers respond to this problem by hiring a vendor, like Dun & Bradstreet, to get clean data. During this work, IT learns they do not understand the business use cases.
Cooper emphasized, “Technology alone does not clean data. It brings consistency and structure by matching similar records.” MDM requires more than this service; it needs a commitment to Data Quality and an implementation aligned with the organization’s culture and maturity level.
Trying to Do It All Yourself
Organizations fall into the pitfalls above and others because they try to do it alone, and most have never done MDM before. Instead, “Organizations have different capabilities with MDM,” said Cooper, “and you don’t know what you don’t know.”
She gave an example of an organization deciding to migrate data as part of a vertical market strategy. That company went with a company that did not have an industry associated with the customer’s data. She added:
“How does an enterprise operationalize a vertical market strategy if it does not know what industry the customer does its business? The company could sit with sales and have that department make the connection. However, that process ends up being subjective and time-consuming.”
Additionally, Cooper talked about two other headaches caused by going it alone. First, Turkey requested the UN to correct the spelling of its name across all its applications.
Also, some of Cooper’s clients became anxious about becoming compliant with business mandates due to the Russia-Ukraine conflict. She advised consulting an expert to bring best practices, an objective data assessment, and understanding whether the data is valid.
Taking Precautions to Avoid the Pitfalls
Companies looking to minimize the three pitfalls mentioned above need to tie their MDM program to their business objectives, look beyond the technology to the data, and work with an MDM expert. Cooper expanded on how to remedy pitfalls during her talk.
Talking With Stakeholders to Align MDM Metrics with Business Objectives
Connecting the MDM program to business objectives requires talking with the stakeholders across the organization, especially divisions with direct financial risks such as sales, marketing, procurement, and supply. Cooper said readers should learn the goals of each unit and how they measure success in growing revenue, reducing cost, mitigating risk, or operating more efficiently. See Figure 2 below:
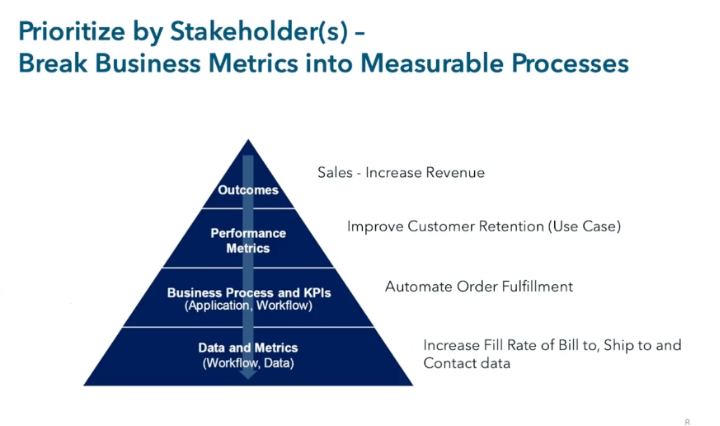
Figure 2 (Image Credit: Amy Cooper)
Cooper pointed to sales as a stakeholder to include. In her model, sales functions to increase revenue, either by selling more products or services or keeping existing customers.
She went on to design metrics to improve customer retention. Cooper uses customer satisfaction surveys as a guide, indicating loyalty when customers receive their orders, the right goods, on time, and with the correct address.
In this situation, the organization needed to benchmark customer data’s accuracy, completeness, and fill rate, particularly the bill-to-address, ship-to-address, and contact name attributes. Then, the company could target improvement on these metrics through automation, which impacts customer retention and drives business outcomes.
Looking Beyond the Technology to Data Quality
Cooper advised focusing on Data Quality – e.g., through reference data – rather than technology. In the figure below, a company has data about a client, Emerson Electric, as shown on the left. The tabular data on the right represents the third-party reference data and a single source of truth provided to the company on the left, which rolls up Emerson Electric’s different legal entities.
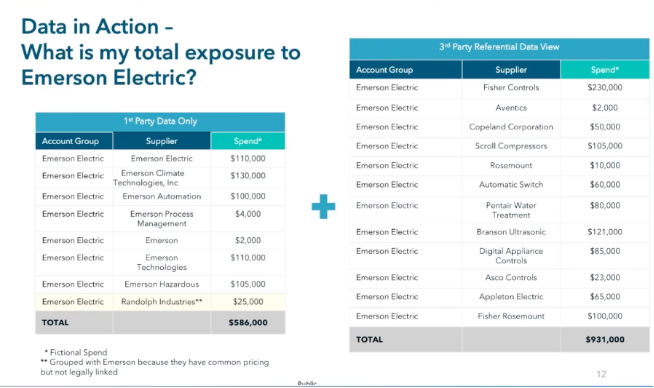
Designing MDM with Organizational Maturity in Mind
In addition to talking with stakeholders and focusing on Data Quality, Cooper suggested designing any MDM program with organizational maturity in mind. She went on to a slide that divided maturity into early, mid-, and late stages and then discussed value creation.
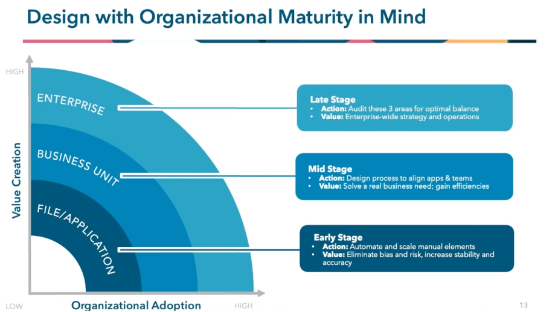
Cooper started at the file/application level on the bottom left and described how she built such a program for a business in the early stage of maturity. The company wanted to create a national accounts program but could not access customer data. So, she identified the customers as the same across the nation based on spend and size of business, and associated this information with their customer relationship management (CRM) application.
At the mid-level, Cooper looks at getting good Data Quality for a business unit and looks to expand this success to multiple teams during late-stage maturity. For example, one of Cooper’s clients started this enterprise-level MDM integration ten years ago.
The MDM integration at the enterprise level has proven difficult because the finance and risk divisions prefer their localized application views. Cooper explained the importance of agreement of teams across an enterprise with how the MDM does visualizations.
Enlisting Expert Advice to Do MDM
As a top precaution, “Talk with peers and people who have done MDM before,” stated Cooper, “and leverage a partner to help with the data.” Such a tactic provides a source of best practices and objective assessments of a company’s data by providing a touchstone for comparison.
She recommended enlisting an expert with accurate and trusted reference data and built-in governance and terminology, providing a single source of truth. That way, an organization can get confirmation about how well their data represents a “valid business entity.”
After a third party evaluates a company’s data, that organization needs to ask the following:
- Where is the data?
- What does it represent?
- Is it accurate?
- Is it available?
- Is it good Data Quality?
She advised organizations to integrate their partner’s data with their own to power “unique needs outside of your business” and get the most trusted views of essential business relationships. In considering this option, companies should dedicate resources to work with their partners on these joint visualizations.
Conclusion
MDM programs can succeed, stressed Cooper. Talking with stakeholders about business objectives, focusing on Data Quality, factoring in organizational maturity, and enlisting experts all minimize impacts from failing to connect business value to the MDM, focusing on technology first, and trying to do it all alone.
She emphasized that engaging in MDM “is a long game,” starting small, improving on MDM activities, and repeating this process to avoid pitfalls. “Target a product, geography, or application to build on MDM successes,” Cooper emphasized.
Want to learn more about DATAVERSITY’s upcoming events? Check out our current lineup of online and face-to-face conferences here.

Watch the DGIQ presentation below: