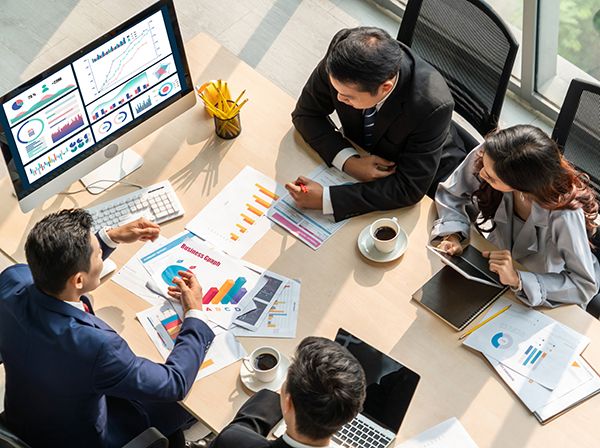
Creating a data maturity model can be useful for a business that is shifting from minimal use of its data to maximizing its potential. A data maturity model is a blueprint that can help determine the best ways to improve how an organization uses its data. It can identify the gaps in a business’s data strategy and promote data maturity within the organization.
Data maturity is not about the age of the data but rather describes the organization’s mature use of data. When a business has low data maturity, it generally means that staff and management are ignoring the value of their data. High data maturity indicates a business is actively gathering and using data to improve its operations. Developing data maturity means creating plans and supporting behavior patterns that maximize the use of an organization’s data.
There are a number of factors that can influence a business’s level of data maturity. The size of a business, the type of industry, the data’s complexity, and the resources it relies on are all factors that influence data maturity.
External factors, such as government regulations, can influence data maturity. Additionally, the organization’s culture and its staff’s understanding of the data’s value can affect data maturity. The tools and techniques that are used are also factors.
Some organizations may see their data as valuable, while others – who use it minimally – may not realize its importance.
What Is a Data Maturity Model?
Data maturity can be considered a measurement system that describes how advanced a business’s data capabilities are. It provides an overview of how the data is, or is not, being used. A data maturity model can allow an organization to assess its Data Governance practices, compare its maturity to similar organizations, and communicate desired improvements to stakeholders. Improving the levels of data maturity helps to avoid poor Data Management and poor Data Governance.
By examining a business’s current policies and procedures, and applying them to the data maturity model, an organization can evaluate its level of data competence.
All businesses are unique, and data maturity models are tailored to fit each business.
The model can be used to identify the areas needing improvement and determine the strategies needed to make those improvements. Businesses can use data maturity models as tools that help determine the organization’s specific flow of data and how it’s being used.
Why Create a Data Maturity Model?
For a business to reach its full potential, its staff must have the appropriate software and place a focus on maximizing the use of their data, while protecting the privacy of their customers. Maximizing the use of data requires gathering and storing data that is accurate – data that is of high quality. The deliberate use of accurate data indicates a work culture supporting data maturity.
Understanding how data maturity models work, and using them to improve a business, makes the business more efficient and effective.
A business could rely on fate and the natural evolution of the business to develop data maturity, but that is a bit of a gamble and certainly a time-consuming process. Creating a data maturity model promotes shaping the organization, and streamlining the evolution of data maturity. The benefits of an organization supporting data maturity are listed below:
- Becoming more competitive: A data maturity model, and the changes it supports, can give a business a competitive advantage and promote intelligent decision-making.
- Identification of goals: A data maturity model can be used as a roadmap to identify the organization’s needs, and help to move it to the next stage of data maturity.
- Improved decision-making: Managers feel comfortable interpreting the data they’ve researched when the data’s accuracy is high. Data maturity supports high-quality data and better decision-making.
- Data literacy: Sharing a common workplace language promotes a form of efficiency called “harmony.” Harmony takes place when there is uniform, expected behavior and a shared common language. Data literacy is an expression of data harmony.
- Data analysis: Data maturity models can integrate data analysis and other automated services into the business’s operations.
When non-management staff members become comfortable accessing and working with the business’s data, they can use the information for their own in-house projects. This happens when an organization has achieved the second stage of maturity. This level of data maturity increases productivity levels and confidence.
Building a Data Maturity Model
Most businesses can use one of these three popular “generic” data maturity models: the Dell Data Maturity Model, the Gartner Data Maturity Model, or the Snowplow Data Maturity Model. Each of these models comes with its own strengths and weaknesses, and one might be a better fit for certain businesses than others. Developing a new model every six months can provide a useful portrayal of how the business is evolving.
The Gartner Data Maturity Model was developed in 2008. It uses five levels of data maturity to represent the goals a business needs to achieve to improve its data maturity. This model places an emphasis on policies and procedures.
The data creation platform Snowplow developed the Snowplow Data Maturity Model. This model describes the different levels as slightly less clunky, and more fluid than the other models, and it is more detailed.
The Dell Data Maturity Model is fairly simple and extremely popular. It provides an easy way to measure how well an organization gathers, processes, and uses its data. The model breaks down each level and defines the steps a business must take to reach the next level. The four different levels of the Dell Maturity Model, and how to attain them, are listed below:
- Data Aware: The first step in developing a data maturity model involves assessing who is using the data, and for what purposes. For example, if the data is being used occasionally as reference material, and reports and forms are created manually, the organization is at level one. Staff are aware of the data but use it minimally.
- Data Proficient: Moving on to level two involves accessing and using automation. Data automation uses software to automate time-consuming, repetitive tasks: data collection, data entry, cleansing, integration, validation, and data analysis. At this level, it would be worthwhile having staff memorize and understand certain key terms and phrases specific to using data (data literacy). Staff have a reasonable understanding of how data can be used and are starting to experiment with “data-driven” decisions and track key performance indicators (KPIs).
- Data Savvy: At level three, the software used for data analytics has been made available, and staff with analytics experience have been hired (or trained). Data is being analyzed and used to develop business intelligence and improve customer satisfaction.
- Data Driven: At this stage, level four, an organization uses data for nearly all business decisions. Automated reports that are based on automatically collected data are used to make data-driven decisions.
Data Governance Supports Data Maturity
Data maturity supports the development of a Data Governance program, and vice versa. A Data Governance program “requires” the use of software and cultural changes. The installation of a Data Governance program promotes the development of data maturity in the following ways:
- The installation of a Data Governance program can significantly improve an organization’s data maturity.
- The trauma of learning a new system – the Data Governance program – creates a shared common framework, acting as a bonding mechanism and building trust as staff and management share in the educational process. A shared educational experience can build trust and develop a new set of cultural norms.
- A Data Governance program also establishes a common language. Different people within an organization may define one word in a variety of ways. However, training provides an introduction to a common definition for words and terms, and a business glossary (part of the Data Governance program’s software).
- “Data Governance’s most significant benefit is coherent coordination among different business areas using the same data and more effective teamwork around that data usage and accessibility,” says Michelle Knight, a researcher/writer for DATAVERSITY. “A company cannot provide the data required to operate without a functioning enterprise-wide governance program.”
Image used under license from Shutterstock.com