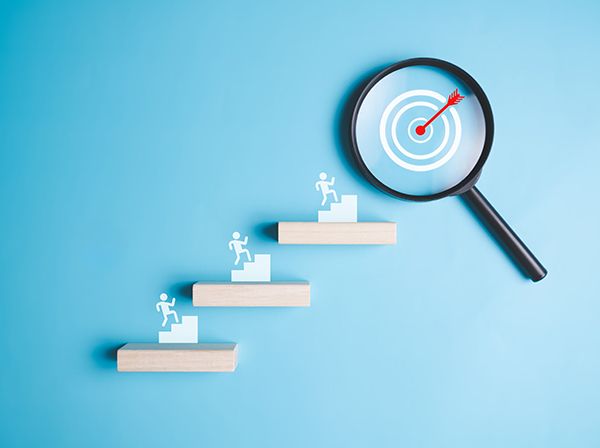
Maximizing the value of data often comes down to ensuring it’s in the right place at the right time and in the right form. While that process may produce magical-seeming results, the road to creating an optimal data quality strategy need not be mystifying. This was the guiding message delivered by Monika Kapoor – director of enterprise technology solutions at Thermo Fisher Scientific – at last year’s Data Governance & Information Quality conference.
As Kapoor sees it, improving data quality is a company-wide project that demands creating a decisive yet flexible strategy in harmony with the grander vision of a company’s mission. Business leaders must then tinker with the implications at the level of functions such as governance and systems.
Almost by definition, such a data quality strategy comprises a nervous system of individual decisions that result in the data processes that build lasting solutions, forge new values, and ultimately, in Kapoor’s words, create “an impact on the lives of whomever we make connections.”
Implementing a Data Quality Scan
Before laying out the groundwork for a longer-term vision for your company’s upgraded data quality protocols, it’s paramount to first perform a sort of “health check” to ascertain what areas of the business stand to be improved. First, management must assess the maturity of the company at an organizational level to predict where new protocols are most likely required. An organization that’s been in operation for over a century under legacy management will be drastically different from a startup with high staff turnover.
Next, data professionals need to locate the most salient gaps in the company’s documentation, whether it’s a matter of glitches in a local area of a customer database or a system-wide failure. Perhaps most important, senior management must create a roadmap of big-picture steps required to strategically obtain the organization’s larger goals.
At every level, it’s vital for any business to have a shared sense of mission in collectively building a culture of data quality that responds to ever-evolving challenges in management at a strategic level.
Senior management must recognize the importance of data to the organization’s mission. This may include data literacy workshops or, for larger organizations, a data roadshow. Kapoor insisted that this step be decisive, but also that it be understood as a process, not a “one-and-done” overhaul, as senior management can grow particularly resistant to change.
Recalibration of goals should play out first in terms of quarterly or yearly objective check-ins, as the realities and personnel of a business may be radically different in the middle term. Otherwise, Kapoor noted, “You are trying to change the fuel in the plane while you are still in the air. And that will be a problem.”
Getting Executive Buy-in for a Data Quality Program
As noted above, the first and most essential step in maintaining optimal data quality is embracing a top-down, holistic paradigm of data health within a company. “Data quality cannot happen sitting in one corner of the organization,” said Kapoor. “Data is like blood. We have 10 interconnected systems in the human body, and if the blood is not right, then your systems will not work properly. Data works the same way.”
In other words, because data systems today are so heavily integrated, the failure of one key data element can bring entire systems to a state of critical inefficiency or even a grinding halt. Therefore, new strategies for ensuring data integrity and reliability must be driven by senior players in management. In Kapoor’s assessment, this can sometimes be a case of teaching an old dog new tricks, recommending that data quality assistance help push through a detailed “SWOT” analysis, or a cataloging of systemic strengths, weaknesses, opportunities, and threats.
Through this sort of “act local, think global” troubleshooting, senior management can successfully winnow only the elements in need of repair, and avoid wastefully over-engineering data quality programs. Presenting a clearly quantified cost-benefit of the process is sure to speak to management in a language they understand.
A second way in which management may need to be coaxed into a new way of seeing is to present a model in which data is not seen only an asset, but as a manufacturing good. “Data is the raw material that goes through different production lines,” Kapoor noted, “and then into the finished code. But data is also a byproduct of the processes: When the processes in the organization go from one step to another, what gets produced out of that data? Data.”
On the flip side, data must be understood as a potential liability as surely as it must be recognized as an asset, as data has the tendency to become dirty as it’s bounced from location to location and among an often-changing cast of staff. Fluctuations in stakeholders, shareholders, and market realities can redefine the value of data, which needs to be seen through the lens of every function of the business. To align so many disparate elements with the long-term mission, Kapoor strongly recommends meticulous upkeep of data dictionaries, training materials, and system documentation.
Data as a Product: A Program for Data Quality Teams
One of the most powerful ways data quality management teams can build a unified systems mission with upper management is to present data as a product in operations – a thing that can be measured and measured again. “Things that you don’t continue to measure can easily spin out of control: like money, like weight,” Kapoor quipped. However, team members need a clear sense of where to target indicative measurement and locate problem areas in the chain of operations. The team needs to have a realistic vision as it makes timetables for improving data projections.
In setting up ongoing data quality metrics that help reveal where data failures recur, Kapoor presented the innovative view that in the end, data is defined by a company’s consumers. “When the data is wrong,” she mused, “who bleeds – the producer or the consumer? The consumer! So they need to become part of the game.”
Just as management needs to steer the ship in a way to implement evolving data needs, data quality teams must communicate with consumers in order to look for persistent ways that data fails them. Such simple inquiries at the consumer level can reveal the faulty cog: For example, customer check-ins might show that when a sales operation specialist was updating orders, they created flaws in the master data and therefore created disasters in UX.
Indeed, measuring experiences at the consumer level can be the most reliable point of assessment in the process of ensuring better data quality. “If somebody is angry, it’s good,” Kapoor suggested provocatively. “They’re frustrated, so try to understand why they’re frustrated, because they want some change to happen. Connecting with your data consumers can address the root cause of the data problem, not the symptom: Close the tap or more dirty water will come out.” By setting up robust avenues of ongoing communication with consumers, data quality teams can not only keep PR and marketing budgets low, but also build trust across all departments in the organization.
Again and again, Kapoor evangelized data quality strategy as a matter of continually calibrating the most humble elements of an organization with its loftiest goals, gauging a forever-competing set of individual needs and priorities against each other as well as the corporate mission. Yet a strategy is in the end a kind of unified organism of administration – it is always more than a single project could ever encompass. “The moment that mindset comes into play, your brain starts rewiring,” explained Kapoor. “Only then will you understand things from a strategic point of view.”
Want to learn more about DATAVERSITY’s upcoming events? Check out our current lineup of online and face-to-face conferences here.

Here is the video of the Data Governance & Information Quality presentation:
Image used under license from Shutterstock.com