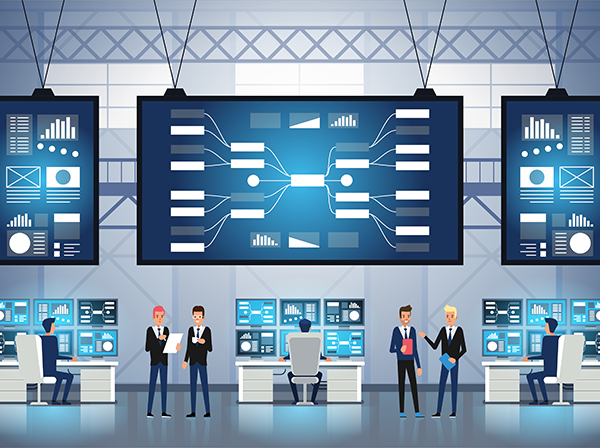
When managers and workers discuss Data Governance (DG), a business program that supports harmonized data activities, vs. Data Management (DM), a comprehensive collection of practices, concepts, and processes dedicated to leveraging data assets, they often use the terms interchangeably. Sometimes, both words point to the same concepts, and these conversations remain productive. At other times, switching between “Data Governance” and “Data Management” leads to miscommunication.
For example, Data Governance, while standardizing Data Quality tasks, does not include technical details about Data Quality cleansing tools; however, this topic does fall under Data Management. So, the mixing and matching terms can create muddled conversations, where Data Governance faces scope creep or Data Management conversations that remain a priority for Data Governance get lost. This miscommunication may explain why 82% of business leaders list confusing Data Governance policies as a top challenge.
One path toward more explicit and straightforward Data Governance policies involves more transparent communications among managers and their staff when using Data Management or Data Governance terminology. This article will sort out Data Governance vs. Data Management concepts to get better and more straightforward outcomes.
What Is Data Management?
Data Management covers all aspects of an organization’s data and how that data is captured, stored, transformed, transported, delivered, used, and deleted. Typically, these activities tie the business strategy and Data Strategy together.
Organizations invest in Data Management to know what kinds of data they have, how to describe it, and why they collect it. This information lends itself to Data Management questions that leaders tend to ask, including:
- Where does the data that we need currently live?
- Can we get our data to where it needs to go?
- Do we have good enough Data Quality so that our data is trustworthy?
To get good answers to these, Data Management requires everyone in the company to work together, from data engineers and modelers to subject matter experts and product managers, especially in cases where each team is responsible for a unique data product. Outside of collaborative efforts, IT, engineers, and others work independently with tools and processes to handle and improve data flow across the organization and in the cloud, doing DataOps activities.
When businesses do Data Management well, through collaboration, they protect data according to business ethics and regulations, making data accessible for business operators and operations. These companies demonstrate their success with the people, processes, and technology available.
What Is Data Governance?
Data Governance is a service under Data Management that connects different components for better integration and legal compliance. While automated tools help and are necessary, they do not define Data Governance. Instead, Data Governance is a program coordinating the enterprise’s people, technology, and activities.
As in Data Management, multiple roles work together in Data Governance. While IT has some representation in Data Governance for guidance and input, businesspeople take the authority in the Data Governance function.
A cross-representation of each team or department agrees on data roles, like stewardship and processes, and assesses how well these activities support the organization’s Data Strategy. So, human behaviors across the organization and around data play a big part in Data Governance.
While Data Governance simultaneously covers Data Management, Data Governance takes a particular Data Management approach, making it suitable for these questions:
- Do we have good enough Data Quality to make our data trustworthy across the organization and different teams?
- Where does our data come from, and where do we store it?
- How compliant are we with current data regulations, and what do we do if there is a data breach?
Similarities Between Data Governance and Data Management
Conceptually, Data Governance and Data Management overlap quite a bit, as Bob Seiner, president and principal of KIK Consulting & Educational Services, mentioned in a 2023 webinar. Both terms focus on Data Quality, integration, policies, and standards.
Both concepts use Data Strategy as a guide and handle data throughout its lifecycle, from creation, transformation, updating, movement, archiving, and deletion. Moreover, Data Governance and Data Management cover emphasis, scope, levels of abstraction, activities, and prominent roles of an organization’s data.
How Do Data Governance and Data Management Differ?
Data Management covers implementations of policies and procedures that do not fall under the mantle of Data Governance. Mainly, focusing on specific technologies and tools and their applications lies outside Data Governance.
To understand why these Data Management activities and discussions happen outside of Data Governance, consider that Data Governance meetings mainly comprise businesspeople, councils, subject matter experts (SMEs), stewards, and partners without specialized IT knowledge.
While Data Governance members want to remain informed about Data Management at a high level, they do not need the technical details. For example, Data Governance discussions may center around protecting data and creating standards around encrypting data. However, IT staff may take conversations deeper, outside of Data Governance, by discussing what encryption algorithm to use and when, how to customize it through ENCRYPT-CSA, or how big to make the critical size.
By moving the technical details outside of Data Governance, organizations can focus on data-driven culture initiatives, change an organization’s approach towards data, and address other human behaviors without getting bogged down in minutia.
How Do DG and DM Work Together?
Data Governance and Data Management (without Data Governance) work together to achieve common goals and follow a company’s Data Strategy. These approaches complement one another.
To grasp how they do so, look at a sampling of Data Governance use cases. For example:
Improve Data Quality for decision-making and operations: The Data Governance approach creates data cleansing and validation rules for when people use Data Quality tools. Also, Data Governance standardizes definitions of critical data elements (CDEs), guiding workers on how to do Data Quality and maintain it.
A Data Management approach outside of Data Governance covers data profiling and Data Quality checks with automation. Individual departments, teams, or individuals determine when and how to run cleansing and profiling tools to meet Data Governance requirements around Data Quality.
Improve data security and privacy while meeting accessibility needs: A Data Governance approach communicates standards to comply legally with data regulations. Through Data Governance, different business units receive training and services to handle data legally.
In Data Management, external to Data Governance, each team across the unit is responsible for ethically using data and staying informed. Engineers set up automation, like servers, to encrypt data and test their setups for appropriate access and security according to Data Governance requirements.
Integrate data for shared business operations: Data Governance aligns data integration with company policies and procedures. It ensures that different teams can complete their tasks and provides tools such as data catalogs, metadata management, or business glossaries to promote data sharing.
Engineers function outside Data Governance to implement integration infrastructure and techniques, including setting processes to extract, transfer, and load (ETL) data from one system to another across data pipelines. According to the Data Governance guidance, business departments process data for integration.
Summary
Anything considered Data Governance also falls under Data Management. When an organization wants to become data-driven, it needs to engage in Data Governance planning and activities crucial to Data Management, such as deciding on best practices to share data.
While Data Governance guides the Data Management implementations, according to the Data Strategy, it does not apply Data Management activity concretely. Engineers in IT or a product team decide which systems will have the data definitions and how to implement them in the programming, such as in programming comments or specific metadata suggested by Data Governance.
Data stewards choose how to handle and maintain Data Quality in a way that aligns with Data Governance standards. Should Data Governance recommendations block Data Management tasks, those issues need to be reviewed at the Data Governance level to get everyone on the same page.
Combining Data Governance and Data Management work leads to more productive discussions and results. The last thing a company wants is for a Data Governance discussion to devolve into the benefits of the Python language over SQL in accessing data through three systems. Nor does an organization want to skip conversations around critical data processes, such as defining Data Quality rules that can be agreed upon across the organization.
Either tactic leaves Data Management incomplete or increases frustration in Data Governance. So, companies will find crisper communication in keeping with the Data Governance approach and introduce other Data Management topics in alternative contexts when needed, ensuring clearer Data Governance and Data Management plans and follow through.
Image used under license from Shutterstock.com