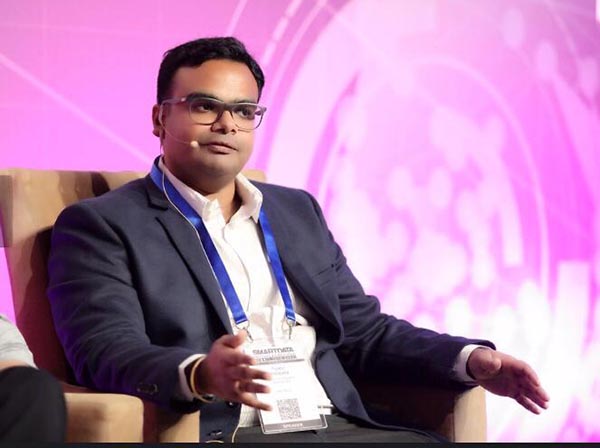
As organizations digitize customer journeys, the implications of low-quality data are multiplied manyfold. This is a result of new processes and products that are springing up. Since the data from such processes is growing, data controls may not be strong enough to ensure the data is qualitative. That’s where Data Quality dimensions come into play.
Increasingly, financial institutions are focusing on data collection management compared to other data stages like consumption, making Data Quality dimensions more important than ever. Among the many factors are recent changes in government policy regarding data privacy and governance, such as GDPR in Europe. In addition to regulatory drivers, this focus on data collection is motivated by the fickle needs of customers, the expansion of digital channels, and the growth of diverse products such as buy-now-pay-later.
The dimensions of quality that a data office has to prioritize for data collection are as follows:
- Accuracy: How well does data reflect reality, like a phone number from a customer?
- Completeness: Is there complete data available to process for a specific purpose, like “housing expense” to provide a loan? (Column completeness – Is the complete “phone number” available? Group completeness – Are all attributes of “address” available?) Is there complete fill rate in storage to process all customers?
- Validity: Is data in a specific format? Does it follow business rules? Is it in an unusable format to be processed?
The use of artificial intelligence (AI) is increasing to generate insights that advance customer journeys. Use cases like credit decisions, personalization, and customer experience are increasingly using AI. The quality of data across the diverse collection of datasets must be assured to reduce the vulnerability of data-driven models.
Banks, for instance, may want to learn more about their customers’ behaviors to better serve them. Typical data points such as customer demographics, “time of usual usage,” and “click streams” can be used in this regard. However, if your organization doesn’t currently have any part of such data, it needs to be collected. Such checks to ensure adequate data is available for a purpose can be formalized as a dimension of Data Quality management. “Availability” is one such dimension that is a one-time check to see if all data is available.
Having been caught in such situations, most data scientists believe that the more data that is collected, the better it is for their analysis. That’s one of the principles behind having to comission lakes with dump-all strategy. On the other hand, data architects might still believe that data can be made available within a short turn-around time for the data scientists, to perform an insight discovery. In this era, where customers are embracing digital capabilities, organizations are transforming their native capabilities to become digitally abled.
Alternatively, you could research the sensitivities and relationships between existing data attributes, and clearly scope the data collection required for the use case. In other words, knowing one’s business purpose and the data being processed is crucial. It is essential to tap into knowledge workers like process SMEs and stewards for this enablement. With a better understanding of the definitions, the time to collect new data can be shortened. Acknowledging this aspect will also allow you to define Data Quality rules that will ensure data integration with consistency.
In financial services, the term “coverage” is used to describe whether all the right data is included for the use cases. For instance, in a lending firm, there can be different segments of customers as well as different sub-products associated with these customers. Without including all the transactions (rows) describing customers and associated products, machine learning results may be biased or can be flat-out misleading. It is an acknowledged aspect that collecting all of the data (often from different sub-entities, point of sale systems, partners, etc.) can be hard, but it’s critical.
- Coverage: Is there adequate population of data for consumption? Does data cover all datasets that provide context to a use case?
To summarize, assessing the quality of information while collecting it first-hand or through alternate sources is important. Moreover, it is crucial to use accurate, complete, and adequate coverage of data for insight generation using artificial intelligence or to avoid process breaks in customer journeys.