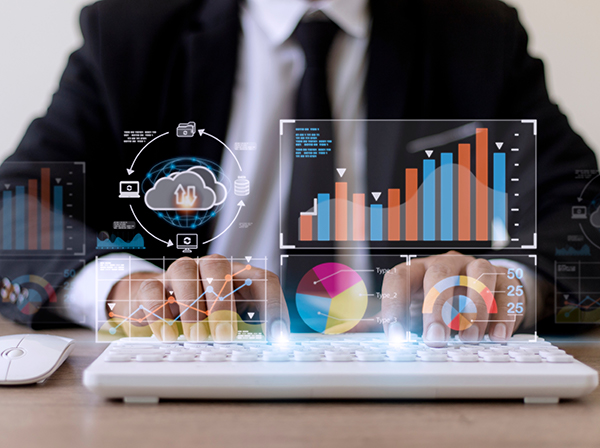
When one thinks of “metrics” in the context of Data Science, the term might denote raw numbers as in descriptive metrics, qualitative labels as in marketing analytics, or comparative labels as in website analytics. Metrics come in many different forms and structures, but the primary objective of Data Science metrics is to measure and report for evaluative purposes.
Metrics, by themselves, may not be very useful, but when used in the right context, they provide meaning to any kind of data analytics activity and aid decision-making.
Here are some examples of metrics used in the context of marketing data:
- Users
- Page views
- Bounces/bounce rate
- Average page rank
- Click-through rate
Another good example of metrics is key performance indicators or KPIs, which collectively indicate the success or failure of an entity. Generally, business KPIs are aligned with broad business goals, and hence become a serious subject of study among all types of business executives.
The Role of Metrics in Evaluating Business Outcomes
In the Data Science world, “data,” “metrics,” and “analytics” mean different things, but they all work together to support business-strategy goals. The whole practice of Data Science is geared toward finding trends in data using metrics – and using that information to support business goals. A modern data analytics solution enables users at every level of expertise to be more insightful in tracking KPIs and to be more accountable for helping achieve business goals.
Data analytics can help you set KPIs and metrics related to the specific functions of your company’s departments: sales, marketing, finance, or HR. For instance, managers in the food and beverage industry are using data analytics and real-time reporting daily to make intelligent business decisions regarding inventory, sales, and customers.
Take the case of Google Analytics. Digital marketers can use at least 14 metrics available on this dashboard that ace marketers can use to make data-driven business decisions. Many marketing organizations use too few metrics for measuring and managing effectiveness, so a holistic performance analysis is not possible.
A common business realm where metrics play a critical role is measuring the ROI of specific programs deployed for achieving performance goals. The ROI metrics indicate the impact of performance goals on actual business outcomes – for example, market share or reviews. Once these metrics are studied in relation to their intended objectives, they help the business leaders decide what to continue doing, what to change, what to innovate, and so on.
How Can Data Science Metrics Help Businesses?
A Data Science team can, for example, measure and evaluate the effectiveness of data-driven marketing campaigns to gauge the real value they add to your organization. These types of measurements help give senior leaders an understanding of what role Data Science plays in the overall business. Metrics, by their very nature, relate to a broad range of data points generated by analytics methods to enable a quicker, granular analysis of marketing efforts.
Data Science, like other business functions such as sales or marketing, uses performance metrics to gauge its effectiveness and performance outcomes. Organizational Data Science teams can develop custom metrics to measure and track different stages in the Data Science lifecycle.
Data science metrics can be very useful in measuring the performance of team members tracked through the various stages in the Data Science lifecycle. One place to look for answers is Data Quality (DQ), and Data Science teams need to design Data Quality metrics around the relevance, accuracy, usefulness, consistency, and other important attributes of data.
An overenthusiastic junior analyst might come up with hundreds of metrics to report back to the C-suite, but until these metrics are clearly aligned to a predetermined set of KPIs, the Data Science reports may fail to deliver. When Data Science projects develop without adhering to a detailed original plan, the random generation of metrics is generally unhelpful. When measuring product success, it is tempting to quickly club together metrics that are aligned with product goals. As a case in point, Shopify found it useful to organize product success metrics in a measuring plan.
It’s important to recognize that Data Science lifecycles, unlike the sales or marketing funnels, generally follow non-linear paths. So, when designing KPIs to measure the effectiveness of Data Science teams, one cannot use traditional metrics to track performance. Rather, one has to assess and understand what performance outcomes are desired within a team and how those map to the entire Data Science project lifecycle.
Data Science tools like an interactive dashboard (instead of a plain spreadsheet) allow professionals to visualize trends, patterns, KPIs, and metrics, and identify ways to optimize each one for profitability. Thus, Data Science tool evaluations through a planned set of KPIs can help identify which features and functions result in maximum results.
Data Science Teams Need to Design Effective KPIs
In the food and beverage industry, business analysts examine sales, product inventories, and financial data to track KPIs accurately. It is important to note that KPIs are critical in helping organizations unlock value hidden within a large data set.
KPIs are most effective when measuring data points that are easy to comprehend, actionable, and highly relevant to your business. KPIs help to demonstrate speed or focus data teams on the things that need improvement. Data analytics KPIs help companies focus on the right insights, so that they do not get bogged down in security logs, vanity metrics, or the never-ending noise generated by the growing numbers of IoT devices.
Data Science with Big Data: Measurements and KPIs
Big data analytics requires more critical thinking skills to locate what is behind the data and use metrics to drive decisions. Measuring ROI from a big data initiative is much more about the business objectives that you are hoping to accomplish, and the metrics and tools used to gauge success, rather than looking at big data as one monolith.
While KPIs are critical for aligning big data analytics initiatives with business goals and outcomes, it can be difficult to identify which ones are most important. As metrics establish the parameters of data your organization will use to gauge performance, the first useful step may be defining an effective set of performance metrics, along with associated data. Also, the metrics must be aligned to the intended performance outcomes.
Conclusion
Chances are, the overall objectives for your organization cover a number of departments and use cases. Each department probably requires a different set of metrics for tracking impact. Once the analytics team has identified the use cases and metrics that are representative of success, it is time to look at how employees are currently using data. When looking at a data project or product, you might have to break your KPIs down to the bare-bones project or product level.
A business needs to look at which skills and training the Data Science team must have to adopt and use the data, analytics, and related metrics for enhancing business performance through data-driven practices.
Image used under license from Shutterstock.com