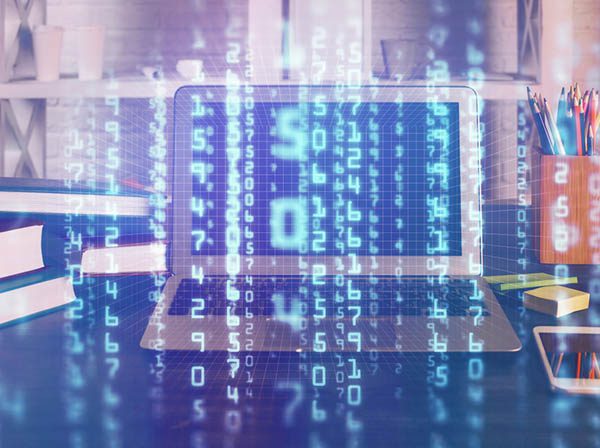
Ask the question “How much Data Quality is good enough?” and see some very puzzled and alarmed looks. Data Quality, comprising all activities making data fit for consumption, plays a fundamental role in trust, security, privacy, and competitiveness. Good Data Quality is critical because it fuels a surviving and thriving business.
While it would be nice to have 100 percent Data Quality for all data all the time, this goal will remain elusive. For starters, companies do not have an infinite supply of money, people, and time. Additional reasons, in-depth, have been listed by Phil Teplitzky in a talk at the Fourth MIT Information Quality Industry Symposium.
However, ignoring Data Quality until an issue arises is not financially viable. Forethought, action, and measurement are necessary. Understanding Data Quality risks, how these impact business processes, and how to proceed given this information will lead to good-enough Data Quality, allowing a business to profit without overrunning time or money.
Webster’s defines risk as the chance of injury, loss, dangerous chance, or hazard. Some risks can clearly be considered ones to avoid. Take, for example, an e-commerce shop that displays entire credit card numbers in electronic or printed receipts. This compromises customer security and violates the Fair and Accurate Transactions Act of 2003, which could result in about $2500 per incident.
Risks can be less clear, and depend on the context. Say an e-commerce business, Earnest Expresso and Trustworthy Tea (EETT), sells expresso whole bean or tea leaf combinations. How would EETT assess the risk in accurately defining flavors for different expresso bean and tea leaf combinations? This information would depend upon the business purpose and on its customer tastes and personal preferences. While this kind of Data Quality seems less risky to consider, it could still sink the business if flavor information is central and also confusing. Not all risks can be considered equal.
Risks fall across a range, from acceptable to business-wrecking. Comparing risk-level scores with measurements across observed results, evaluates how much Data Quality is good enough.
To do this effectively, businesses need to formulate good requirements — decided through Data Governance — defining what adequate Data Quality looks like.
Companies also need to know what Data Quality outputs can be measured and how to do so, as well as how frequently these results can be reproduced. In addition, adequate risk coverage for data inputs needs to be considered. These principles form a basis for scientific inquiry, and factor in determining how much Data Quality is good enough.
Business Requirements Inform and Also Need Good-Enough Data Quality
Enterprises need to construct good business requirements so that technical, operational, and other departments know how to interpret and use data to do their work. Many companies have implicit Data Quality business requirements, such as customer payment data needs to correctly match the sum of all price points of purchased items. This falls under common sense. However, only business requirements at a tacit level confuse what is good enough data.
Standards need to be formalized through Data Governance, a collection of agreed-upon practices and policies. Data Governance specifies, objectively, what Data Quality risks should be acceptable and how this can be measured. Furthermore, these business standards are also data, and must be vetted by Data Governance for Data Quality.
Back to the example of the Earnest Expresso and Trustworthy Tea shop: This business requires unhealthy expresso consumption to be flagged and non-caffeinated tea products suggested instead. Experts’ recommendations for healthy for adult consumption vary from one to four eight-ounce cups of expresso drink per day. If an EETT marketing person chooses to make one eight-ounce cup of expresso drinks per day as a healthy threshold, but the operations department puts the maximum at four eight-ounce expresso drinks, then who is correct? What amount of risk is OK? Arguments could subjectively and accurately be made for either case, but one value needs to be chosen. Multiple values will compromise Data Quality and cause confusion. Data Governance steps in here and calls for an agreed-upon objective maximum value for expresso consumption. Data Quality becomes good enough with everyone in EETT and consumers in alignment about the good objective measure.
Data requirements also need to be re-reviewed for completeness by Data Governance to achieve good enough Data Quality. Say the EETT decides to expand its market from the US to North America. EETT’s specification states that the product is priced by weight with a correct measure. Would that be adequate? The types of weight measures differ in the US from Canada and Mexico (ounces vs grams). So, if EETT details expresso bean and tea leaf products on its website, how does the developer know how to convert the value so customers in New York City vs Toronto can see the correct number. The outputted data needs to be converted and the requirement is incomplete. This increases the amount of risk substantially for unacceptable Data Quality. To ensure Data Quality is good enough, business standards have to be complete.
Good Enough Data Quality Means Reproducibility with Acceptable Risk
Well-formed requirements need reproducible good Data Quality to assure results meet acceptable risk. Furthermore, as Victoria Stodden from the Department of Statistics at Columbia University states, good enough Data Quality comes from recreating these outcomes within the same available computer codes and data sets parameters. Say the EETT requires monthly sales reports. Reproducible Data Quality means regardless of which day the November 2019 monthly report is run, or how many people run the report, the same results should appear, as long as the same computer code and data sets are used. This is what business needs for Data Quality to be good enough and risk to be considered acceptable.
Given this definition of reproducibility, complexities occur in interpretation, as the same data set is not always used and everyone might not be aware of this. For example, an EETT salesperson generates a monthly report for March 2020. On March 10, March 17,, and March 27, a person generates a report but the number of sales and profit differs. Does that mean Data Quality is not good enough? Not if the report spans “month to date.” It would not be possible to know on March 10the sales and profits to be made on March 27 (unless a future oracle appears). The same report has been run with different data sets.
Also, using different computer code can confuse risk assessment and whether Data Quality is good enough. In these cases, one-time observations can be considered poor Data Quality. Say EETT did not see a credit card transaction from a purchase, but the financial institution recorded the charge the next day — then would Data Quality be an issue for EETT? Yes. The bank’s code correctly processed the transaction and has been validated. The EETT does not have the same code to send the purchase to the bank and needs to fix this to integrate with the bank’s system. EETT’s failure to process that purchase, in this situation, means its Data Quality needs to be improved.
Good Enough Data Quality Needs Adequate Coverage of Data Inputs
Generating good requirements, vetting them for Data Quality and ensuring reproducibility needs to be combined by covering data inputs. As Tejasvi Addagada notes, the right data used as inputs needs to be identified. This “right data” changes with time and business priorities. This is especially important in machine learning, algorithms that parse data and make a determination or prediction about the world, and affect whether Data Quality is good enough to meet adequate risk.
Take the following example. Earnest Expresso and Trustworthy Tea creates a machine learning algorithm to learn what type of payment has been made — credit card, debit, cash, etc. — to better handle multiple refunds, give discounts for products, and to learn customer preferences. But when customers use Google Pay or Apple Pay, many transactions are tokenized, and the payment type is not known by EETT. How can EETT cover the correct payment type inputs so that the machine learning algorithm runs successfully? Here, Data Governance needs to investigate and combine business and IT knowledge, figuring out how to achieve adequate payment type data input coverage for a reasonable risk level. Once this is known, the company will find it easier to develop and use a good quality machine learning program.
Data Quality remains critical to a business, but it has to come with reasonable costs for a business to be viable. Companies do this by assessing risk and comparing this to observation. Requirements, reproducibility, and coverage provide tools to do this comparison and get good-enough Data Quality.
Image used under license from Shutterstock.com