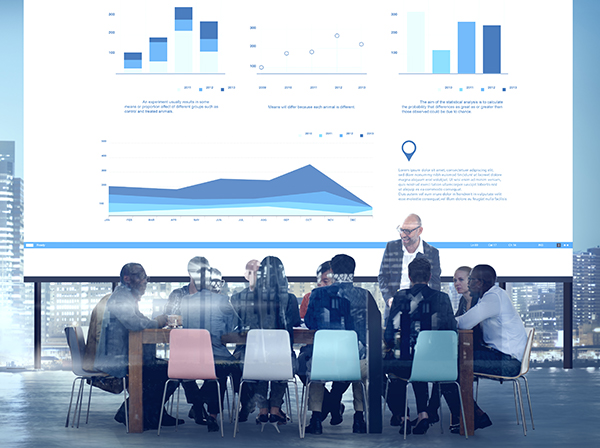
When most people think of analytics, they tend to picture a rigidly left-brained data scientist more at home with computer programming than creativity. But analytics leader and author John K. Thompson challenges such a notion. During his keynote presentation at DATAVERSITY’s Enterprise Analytics Online event, he proposed instead that the members of an analytics team can be as much artisans as scientists – if not more so.
Drawing from his 30-plus years of experience in business intelligence and advanced analytics – working within 20 different industries – Thompson shared battle-tested techniques for creating, building, and managing successful analytics teams.
Data Artisans, Data Workers
Enterprises typically take one of two main approaches when building an analytics team, said Thompson. The first approach, which he called the “artisan model,” involves building a small team of highly experienced (and highly paid) data scientists. Such skilled and capable team members can generally tackle all aspects of solving a business problem, from subject matter expert engagement to hypothesis testing, production, and iteration.
The “factory approach,” on the other hand, resembles more of an assembly line, with a large group of people divvying up tasks based on their areas of expertise: some working on the business problem definition, others handling data acquisition, and so on. This second approach requires hiring more people than the first approach, but the pay differential between the two types of team members is significant enough that the two approaches cost roughly the same.
Although Thompson personally prefers the artisan model – he tends to “resonate with people who are going to take accountability, responsibility, and run with something from the beginning to the end” – he advocates for the value (and, to some degree, necessity) of implementing both methods. With this third, hybrid approach, an elite team of artisans leads the way, farming out the repetitive elements to a third party, using automation wherever possible and advisable.
Which approach works best? There’s no one-size-fits-all answer, as every organization differs in its management style and workload. What’s more, success may lie less with your choice of model than with a proper understanding of the role of data science:
“A data science team is not the same as a development team,” noted Thompson. “In a data science environment, you’re really exploring, you’re trying to drive innovation, you’re trying to drive change in the organization. And that really takes a level of creativity, and a level of exploration, more akin to artisans.”
All that exploration and creativity can require lots of flexibility in projecting timelines, as the incubation process – the analogy to the artisan applies here – does not work on a predictable, linear timetable. “That can sometimes be hard for people to understand and grasp because at first, they’re not used to dealing with data-oriented teams,” explained Thompson. “It’s very difficult to tell people, ‘It’s going to be three months, six months, a year,’ because sometimes we don’t know if the problem can even be solved.”
Analytics in Real and Possible Worlds
The way Thompson sees it, building real-world analytical processes brings together “two different worlds driven by two different forces.” On one side, data scientists are immersing themselves in analytical concepts – designing, testing, and building analytical models driven by the “art of the possible” – while on the other side, production managers are bringing them back to earth by focusing on practicalities such as compliance with regulatory requirements.
The relationship between these two sides depends on the leadership that connects them: If a team of data scientists can be seen as the left and right hemispheres of the brain, strong analytical leaders serve as the corpus callosum, drawing out the strengths of both in a smoothly symbiotic flow. Often, analytical leaders struggle to serve as liaisons or even translators between the visionaries and the boots on the ground. “Sometimes, people on each side don’t quite appreciate the requirements and the rigor and the diligence that’s required on either side of the equation,” said Thompson.
Therefore, he encourages analytical leaders to spend time with the executives who govern each phase of production and clarify what each expects from the analytics team, whether it’s a simple number or a robust process. In this way, execs are given a glimpse of the full menu of possibilities and can choose the most suitable options based on their preferences.
In Thompson’s experience, it is in this part of the process that the biggest problems arise, “not in the analytical concept side, or acquisition of data, or modeling or the math, but when analytical leaders are not communicating as clearly as they need to the business leaders.”
Daily Operations of an Analytics Team
Even if the analytics and production teams strike a harmonious balance of operations, changes in the outside world can still cause disruption. Management must accommodate these external factors by continually recalibrating analytics cycles – retraining and updating models on short notice to meet new business requirements.
Some businesses can expect a reliable lock-groove of operations, with a one-year “analytical cycle” that allows for leisurely manual tinkering. On the other hand, a company contingent on mercurial fluctuations – such as real-time credit risk analytics – may have to contend with cycles of only a few hours and lean heavily on automated solutions.
Translating these shifting demands into daily operations ultimately boils down to assigning clearly defined tasks to data scientists in real time. To accommodate the unpredictable, Thompson devised a “personal project portfolio,” a sort of conceptual dashboard that coordinates all projects great and small in a data scientist’s workload.
A personal project portfolio creates a pipeline that will fast-track items of lower priority to the forefront of operations when real-world obstacles put more pressing demands on hold or are canceled altogether. This dynamic mix of projects benefits not only the business, but the data analytics team as well.
“I always think of data scientists as having Ferrari brains,” quipped Thompson. “They’re always running at high idle. So, you want them to be able to time-slice between different problems and different challenges to keep them engaged. The portfolio takes care of that.”
The Ultimate Balancing Act
An analytics team needs to grow and evolve to survive, and management must treat its staff accordingly. “Data scientists are some of the most sought-after talent in the economy right now,” Thompson stressed, “So I’m working every day to make sure that my team is happy and that they’re getting work they’re interested in – that they’re being paid well and treated well.”
All the while, analytical leaders must perform a perpetual balancing act between two paradigms that, in optimal situations, are complementary yet discrete. “We’re grafting this creative, artistic process onto a very mechanistic one,” he explained. “Synchronizing the needs of the production refresh cycle with the modeling team’s ability to deliver is the key to success.”
Want to learn more about DATAVERSITY’s upcoming events? Check out our current lineup of online and face-to-face conferences here.

Here is the video of the Enterprise Analytics Online presentation:
Image used under license from Shutterstock.com