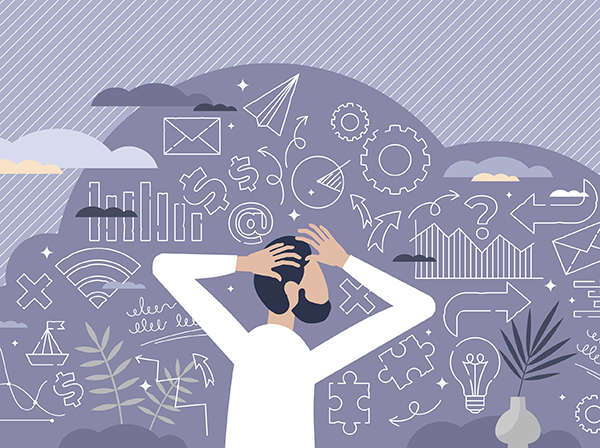
With so many different ways that data can be created and collected within an enterprise, it’s no surprise that Data Management challenges abound. Data usually comes from various sources, some of which cannot be trusted to be safe and compliant with regulatory standards. It can be gathered from large numbers of sources such as smart devices, sensors, video cameras, social media, and so on. Then comes the problem of storing massive amounts of data and integrating them for further use. Finally, the stored data must be cleaned and prepared for analysis.
The primary goal of Data Management is to assist individuals, businesses, and related entities in improving their data usage within the limits of Data Management strategies and regulations. When Data Management systems are maintained properly, data can give businesses valuable insights into their operations, supply chains, customers, and future business prospects.
The use of data is becoming more critical for businesses around the world in managing day-to-day operations and for making business decisions. However, enterprise Data Management systems come with many visible and hidden risks and challenges.
Common Enterprise Data Management Challenges
Data Management helps minimize potential errors by setting up processes and policies for data use. Moreover, a strong Data Management strategy creates confidence in the data used for organizational decision-making.
Once you establish a Data Management system to ensure that data is trusted across the organization, the built-in processes are expected to improve the quality of the data. The most common challenges around Data Management involve entering and organizing the data and having appropriate processes for using that data.
The second Data Management challenge is the data silos in your organizations. Inconsistent or incomplete data sets across departments can easily lead to bad queries, resulting in performance issues and lost time, resources, and money.
Without data standardization, your organization can run into integration issues across databases. The most common challenge data-driven organizations encounter is keeping various systems in sync with each other. Another closely related challenge is integrating massive amounts of disparate data into a central data platform; this lack can make it difficult to accelerate data-driven decisions.
The third challenge is managing the data spread across several locations, where business units are using hundreds of enterprise-level applications. Storing and accessing massive amounts of data presents challenges when teams are tasked with managing the security, reliability, and uptime of several databases within a hybrid IT environment, in addition to their daily tasks. Large companies can have dozens of enterprise solutions, each with their own data warehouses, like CRMs, databases, ERPs, etc.
The fourth challenge is managing data across multiple countries or currencies, when large businesses have multilocation business units that do not necessarily work in concert. Additionally, these multinational businesses often use data-technology platforms or tools that do not use data in a compliant manner. It is common for such organizations to face issues with data security and poor Data Quality. The Data Quality problem can get compounded with Data Governance (DG) and analytics teams trying to extract divergent types of data.
The fifth Data Management challenge is managing big data. With big data, the data storage issues, though significantly reduced with cheap storage solutions, are not over yet. Handling big data, by its very definition, usually involves a lot of data stored across different systems and platforms. When diverse types of databases fail to communicate with each other, the result can be a mega Data Management mess. The biggest challenge is, of course, combining extremely large data sets from CRM and ERP systems, as well as other data sources, into one single, easily managed big data architecture.
The sixth and last Data Management challenge included in this article is related to the newer
cloud data management, which includes a set of challenges beginning with data security and ending with real-time analytics.
Probable Solutions for Tackling Data Management Risks
Automation: Today’s leading Data Management software helps with everything from preparing the data, cataloging, searching, and management, making it easy for data managers to find the information they need quickly. Data catalogs help with metadata management, creating an overall picture of data, providing summaries about its changes, locations, and quality, as well as making data easier to locate.
Data Quality Policies: The ultimate goal of Data Quality (DQ) policies is to provide data in a form where it is ready to be analyzed and processed by other tools. Data Governance can improve visibility into the data assets in an organization, making it easier for individuals to find the correct data quickly and with confidence for their analyses.
Data Governance Measures: Data Governance (DG) plays a key role in tackling Data Management challenges. In spite of the inherent risks involved in bad data ethics and Governance, many companies do not take the steps necessary to provide adequate DG measures. Poor risk management, data breaches, data silos, lack of compliance, and an unregulated environment – these are all potential challenges related to governance failures.
Advanced Databases: Using high-scale, high-performance databases helps the organization analyze data from various sources using machine learning and advanced analytics. By using unifying analytics platforms for measuring and marketing, organizations can overcome the challenges in managing data, freeing up human talent for deep analysis.
Lessons on Data Management
For those business users who keep up with industry literature and follow thought-leadership articles on tackling Data Management issues, here are some pearls of wisdom.
According to Gartner, with business decisions becoming “more connected, contextual and continuous,” it is high time the entire process of decision-making was reinvented. This is where adaptive AI systems come into play by enabling quicker and more environment-sensitive decisions. The adaptive AI systems require sound AI engineering practices, which makes applications highly responsive to change.
Gartner also recommends that AI-powered Data Management systems need to focus more on data. Without data-centricity, the AI systems can become risky and useless. In data-centric, AI-powered Data Management systems, issues related to data integration, data bias, data labels, or metadata are handled in a strategic manner.
The data fabric learns from the data and then “acts on the metadata.” By making good recommendations for processes and people, data fabric actually reduces a substantial portion of the Data Management tasks involved in designing and deploying a robust Data Management system.
Here is a Forbes post distilling the meat of an interview with Taylor Culver, founder of XenoDATA. According to Culver, the struggles of data leaders are very similar to the struggles faced by human communicators, storytellers, or collaborators; the positive outcomes are always led by people. Thus, the role of the human network is critical in enterprise Data Management.
Image used under license from Shutterstock.com