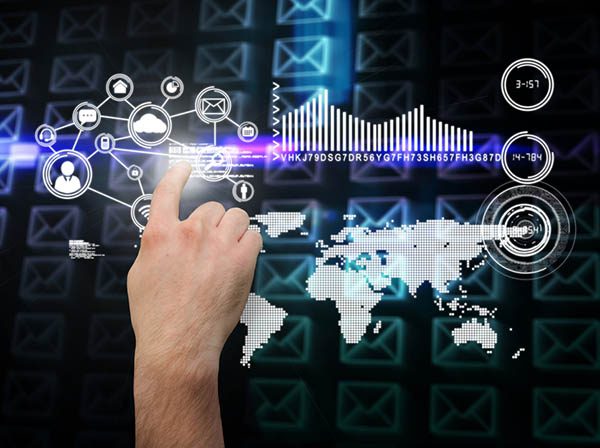
A Data Engineering Guide reveals that while people often rely on the work of data engineers — depending on Siri for quick solutions or being enchanted by custom recommendations or promos — they often do not realize that these advanced tools can provide accurate results only because of the hard work put in by data engineers. The analysis conducted on big data showed it is only as good as Data Quality, which is prepared by data engineers. According to this guide, “Data Engineering is the act of collecting, translating, and validating data for analysis.”
So in an ideal world, the data engineer sets up the data warehouse, the data framework, and the data pipelines for the data scientist to conduct complex analysis on. They work together in harmony but never step into each other’s role.
The article The Changing Data Science And Data Engineering Tooling Environment warns readers about the danger of interchanging Data Science and data engineering roles in an organization. Like all other technology fields, data technology fields ought to maintain sharp distinction between “specializations,” which is why professionals with pure Data Science skills or experience should never be expected to fill in the role of a data engineer and vice versa. The message in this article is clear: Data Science and data engineering are vastly different activities that require skill sets suited for only one role or another, but not for both.
So You Want to be a Data Engineer? points out that while data scientists set their focus on big data analysis, the data engineers set up the data architectures and the data pipelines for the data analysis to take place.
Especially now, with the integration of AI and ML with data technologies, data engineers are vested with readying the data pipelines while the data scientists strictly perform data analysis to extract insights. Thus, the data engineer’s role has become as important, if not more, as the role of a data scientist.
To a large degree, future automation tools may minimize the need for data engineers, but they will still play a significant role in enterprise analytics teams. The problem with modern tools vendors is that very often they offer technology environments for data scientists, which actually require data engineers to be present and perform the initial data setup, cleaning, and preparation tasks. Let’s hope that with time, this separation of roles will become clearer to business leaders and operators.
The Evolving Role of the Data Engineer
With rapid technology advancements, data engineering, as a practicing field, is headed for complete transformation. The current developments in data engineering have been impacted by Internet of Things (IoT), serverless computing, hybrid cloud, AI, and machine learning (ML).
The Emergence and Future of the Data Engineer points out that the wide adoption of big data led to the birth of the data engineer. However, the biggest change in data engineering has happened in the past eight years, and that due to rapid automation of Data Science tools.
The modern business analytics platforms come equipped with fully or semi-automated tools that collect, prepare, and cleanse data for the data scientists to analyze. Nowadays, the data scientist does not have to depend on the data engineer to provision the data pipeline as they once did several years ago.
In this scenario, a single data engineer is sufficient for supporting an entire team of five or six data scientists/analysts. The data engineer is still required to tweak the data infrastructure and enable the team members to work more efficiently, but advanced automation technologies are reducing the need for data engineers. Or are they? The Role of Data Engineer Is Changing gives a deeper understanding.
A feature article indicates that global businesses often “struggle” to move from legacy data to a “more flexible architecture.” This is where the role of a data engineer becomes critical for the digital preparedness of a business. Eighty-five percent of survey respondents of a recent McKinsey survey reported that they were “somewhat effective in meeting their goals for their enterprise data and analytics initiatives.”
Jasmine Tsai, Director of Engineering for Clover Health’s Data Platform, shares her experience as a data engineer in the blog post The Future of Data Engineering is the Convergence of Disciplines. She reflects that the “data engineering . . . in the near future . . . (will) overlap with other fields, especially software engineering.” Tsai comments that very soon, data engineering may resurface as a hybrid role.
In Data Engineering Trends for 2019 revealed that “real-time analytics, streaming analytics, near real-time analytics, complex event processing” are technology trends applicable to both data engineering and software engineering. So essentially, there are layers of overlap between software engineering and data engineering.
Today’s data engineers are not only well versed in all types of cloud environments but equally conversant with technologies “ranging from Internet of Things (IoT) to Logical Data Warehouses (LDW).”
The Future of Data Engineering
With the move from batch-oriented data movement and processing to real-time data movement and processing, there has been a significant shift toward “real-time data pipelines and real-time data processing systems.”
The data warehouse, with its tremendous flexibility to house data marts, data lakes, or simple data sets based on need, has become very popular lately. Emerging Trends in Data Engineering explains how database streaming technology is preparing the future of highly scalable, real-time business analytics.
The following four areas have been earmarked as technology shifts in data engineering of the future:
- Batch to Real Time: Change data capture systems are rapidly replacing the batch ETL, making database streaming a reality. The traditional ETL functions are taking place in real time now.
- Increased connectivity between data sources and the data warehouse
- Self-service analytics via smart tools, made possible by data engineering
- Automation of Data Science functions
- Hybrid data architectures spanning on-premise and cloud environments
Another impactful shift in data engineering technology in the recent times has been to see “data as it is” rather than worrying about how and where it is stored. The shift to real-time data processing has made data access painless and data processing more challenging. Data Management Trends in 2020 throws the spotlight on the Enterprise Data World Conference, where many distinguished speakers will be present to discuss their personal experiences related to data engineering.
Closing Notes
The webinar titled Data Quality, Data Engineering, and Data Science offers a discussion on the creation of a data lab for developing “the right data standards, patterns and principles,” and a data factory for implementing such standards. The future data engineer will take an active role in fulfilling the demands of both the data lab and the data factory.
Image used under license from Shutterstock.com