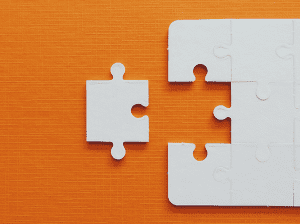
Data completeness is an important aspect of Data Quality. Data Quality is a reference to how accurate and reliable the data is overall. Data completeness specifically focuses on missing data or how complete the data is, rather than concerns of inaccurate or duplicated data. A lack of data completeness is normally the result of information that was never collected. For example, if a customer’s name and email address are supposed to be collected, but the email address is missing, it is difficult to communicate with the customer.
Additionally, for data analytics to function properly, a high level of data completeness is needed. A major problem with resolving incomplete data issues is the lack of software. Currently, missing information must be filled in manually.
Missing chunks of information restrict or bias the decision-making process. Attempting to perform analytics with incomplete data can produce blind spots and biases, and result in missed opportunities. Currently, business leaders use data analytics to make decisions that range from marketing to investment strategies to medical diagnostics. In some situations, data missing key pieces of information is still used, which can lead to dangerous mistakes and false conclusions.
Assessing and improving data completeness should be done before performing analytics.
Examples of Incomplete Data and Their Consequences
A simple example of how a lack of data completeness would damage profits can be shown by the absence of key real estate details, such as square footage. Without this information, an appraiser cannot accurately evaluate the property’s value. Attempting to appraise the value of an apartment, a home, or even undeveloped property would be clumsy at best, and potentially disastrous. Estimating the costs of any project without measurements could result in disaster.
Incomplete consumer data offers another example of how a lack of data completeness can damage profits. Generally speaking, consumer data is not considered complete unless all the requested data has successfully been filled in and stored properly. For example, having only a name and home address doesn’t help with marketing emails. Missing data can block communications with potential customers. Other potential problems caused by a lack of data completeness are listed below:
- Operational efficiency: The use of incomplete data can damage operational efficiency. A lack of complete data in supply chain management or inventory can cause disruptions and delays.
- Customer insights: Incomplete customer data can produce a limited view of the customer’s behavior and preferences. This can result in irritating a customer or even insulting them. When businesses operate with an incomplete story, the missing info can create misconceptions about the customer’s preferences, market trends, etc. Gaps in the customer’s data can hurt the ability to personalize and target specific customers.
- Regulatory compliance: Several industries are now subject to regulations requiring accurate and complete data reporting. A lack of data completeness can result in fines, legal issues, and reputational damage. Additionally, missing transactions can cause under-reported revenue, in turn causing tax problems.
- Forecasting and planning: When incomplete, historical data, which is often used for forecasting and planning, can have a significant negative impact.
- Machine learning: Data completeness is necessary for developing training machine learning models that function efficiently. Missing data can cause biases and reduce the system’s predictive accuracy.
- Strategic insights: Organizations rely on data completeness when researching marketing opportunities, assessing risks, and optimizing operations. Complete data is needed for strategic planning.
- Effective decision-making: Complete data is essential for making informed decisions. Having access to all the relevant data supports better decision-making.
- Accurate analytics: The use of incomplete data can corrupt a data analysis. When critical data is missing, it can skew the results, making invalid conclusions a high probability.
Analytics and Data Completeness
Any statistical analysis that is based on data with missing values has an increased chance of being biased. Data completeness, as a part of data analytics, is essential when developing a model. The data collected for the research should cover the scope of the question being researched. Any gaps, missing values, or introduced biases will impact the results.
Data completeness is necessary for any organization that relies on data for research and decision-making.
Ensuring Data Is Complete
Data completeness significantly impacts Data Quality and supports good communication with customers, co-workers, and other computer systems. It is important to prioritize and resolve data completeness issues as they arise.
Taking the following steps can prevent incomplete data from entering your systems:
- Decide what information is critical: When forms are used to gather information, some fields are necessary for doing business, while others are not. The fields that are critical to analytics should be identified.
- Make certain fields a requirement: Some people automatically assume a phone number is a requirement, but in purchasing an item from a website, how often is a phone number actually used? A name, shipping address, email, and credit/debit card number are necessary, but any additional information is for marketing or research.
- Use data profiling: Data profiling can be an important aspect of data preparation for processing and analytics. Data profiling is the process of examining data to determine its overall Data Quality. Additionally, data profiling includes a review of source data. (Source data can be useful in backtracking to find the missing data.)
- Assign responsibility to an individual or a team: Have a dedicated individual who is responsible for data completeness. A team could be made responsible for Data Quality as a whole.
- Using the right data source: Only trusted data sources should be used. These sources must place an emphasis on Data Quality, accuracy, and completeness.
Regulatory and Compliance Risks
While profits are often considered to be the primary goal of many businesses, these same businesses are required by law to meet certain standards. Many organizations are governed by strict regulations mandating complete and accurate reporting. Should a business provide incomplete data, it may face charges of non-compliance, resulting in penalties and legal complications.
A casual attitude toward regulations and online business laws may cause more damage than simple financial penalties. A legal misstep can damage a business’s reputation. Damage to a business’s reputation can in turn make attracting new customers a challenge.
The Lack of Software Tools for Data Completeness
The lack of software available for data completeness shouldn’t be surprising. Consider that correcting the spelling of a word or name is commonplace, so improving Data Quality by correcting data is not difficult. Seeking out duplicated data isn’t difficult for the right software either.
But filling in a blank? What do you put in the blank space? If you had the information readily available, this wouldn’t be a problem. Instead, your only hope for filling in that blank requires time-consuming research. And a software program or AI will have exactly the same problem.
There are a few software programs available that work with specialized research that uses highly standardized information. These programs “predict” what the missing information should be. Sadly, even with highly standardized information, mistakes can be made, and a human should review corrections.
The Future of Data Completeness
The most efficient method for filling in the blanks is to do it with the customer, or at a time the information is easily available. “Required fields” is perhaps too simplistic a solution, as it can block sales transactions if the potential customer is lacking, or unwilling to share, a required piece of information.
A partial solution would be software that seeks out and identifies missing information within the data, then provides its location.
ChatGPT may be a possible solution, performing the research needed to fill in those missing pieces of information. A concern with this solution is that the resulting research would have to be double-checked by a human (still less time-consuming than doing the research yourself); ChatGPT has developed a reputation for being imaginative and creating the answers to questions when it can’t find an answer.