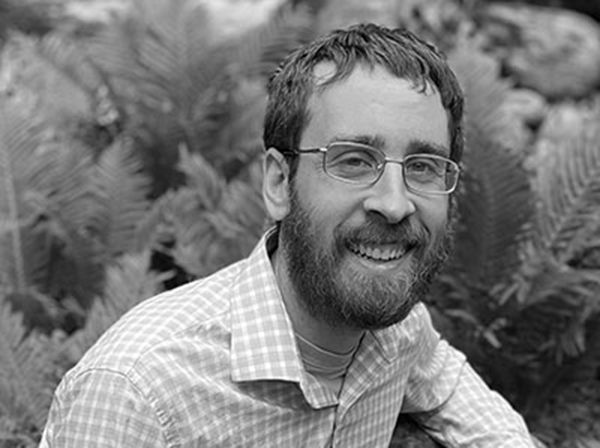
“The democratization of data science” or the “democratization of AI” have long been popular buzz phrases. “Citizen data scientists” poke through open-source datasets finding valuable insights and sharing them with the world out of an individual sense of curiosity. And although this absolutely does happen, the reality is most of the time these democratized advances have not been done by random individuals, but by professionals in their free time. It is a freedom of one sort – not corporate analysis chasing after profit – but not truly democratized in the sense of a curious novice advancing the field on their own.
AI has seen many attempts at low-code solutions that anybody can use. But working in this field for 15 years, I have realized that doing it yourself is rarely that simple. You can pull a standard dataset and run standard algorithms on it – certainly. But every real-world project I’ve participated in has been painful in complicated and trying ways. The data doesn’t join up quite right. The annotators can’t agree. The test set is different from the training set in an important and fundamental but hard-to-detect way. And a finger’s breadth below the surface of most friendly AI systems is an immense amount of math and jargon. Following the beaten path, you can ignore this. But setting out on your own, it has a way of rearing its ugly head and demanding your patience and learning. That’s not to say you can’t teach yourself along – I worked in this field as a professional for many years as I frantically taught myself what I was doing – but it’s always fallen short of any broad accessibility worthy of the moniker “democratized.”
Until, I would say, now. The AI Art field (e.g., Dall-e and Stable Diffusion where deep networks turn free text descriptions into pictures) is filled with cute tricks – appending “trending on ArtStation” in order to convey to the model that you don’t want an ugly picture, but rather one worthy of viral sharing. Artists – the cultural antithesis to us mathematicians – are cranking out involved studies on how dozens of camera names affect the final image quality. Amateurs are learning and sharing and teaching and creating beautiful and baffling creations.
ChatGPT, in its quest to find likely continuations to what you typed, is constantly revealing new capabilities via a clever prompt. An early one was the observation that “tldr;” induces a summary of a document. Understanding that the core algorithm in language models is the prediction of a likely continuation it’s clear why that capacity would exist. But it’s clever to find it. Although ChatGPT struggles immensely with math, coaxing it with “show your work” or “let’s work it out” causes it to write out the intermediate stages in a calculation, which it then attends to while solving the math problem now successfully. These and many more capacities were discovered. And although frequently the discoverer is a professional in the field, it’s no longer a requirement.
Tons of highly complex, professional work is still going on in AI. Not every problem can be overcome with a clever prompt. And the computation required for a ChatGPT or a StableDiffusion precludes it from being the right tool for every problem. But there’s a new space growing for working with these systems in a powerful, creative way that does not demand programming expertise and a deep familiarity with the field. I’m excited to see how this blossoms within organizations where the potential of data science has been too frequently locked behind scarce and overcommitted resources. This is not to say a novice can safely wield these technologies in a commercial setting – we’ve seen again and again how much damage poorly thought-out AI programs can do, either in digital brand mascots corrupted into racism or in the amplification of biases and errors in the training data. But at a minimum, there’s suddenly this expanding region of space in the field that demands play – experimentation and lateral thinking and intuitive understanding of the behavior of these artifacts as opposed to their technical construction. In the weird prompted antics of ChatGPT and the bizarre and beautiful Stable Diffusion artwork, I finally see what I’d call “democratization” in our field.